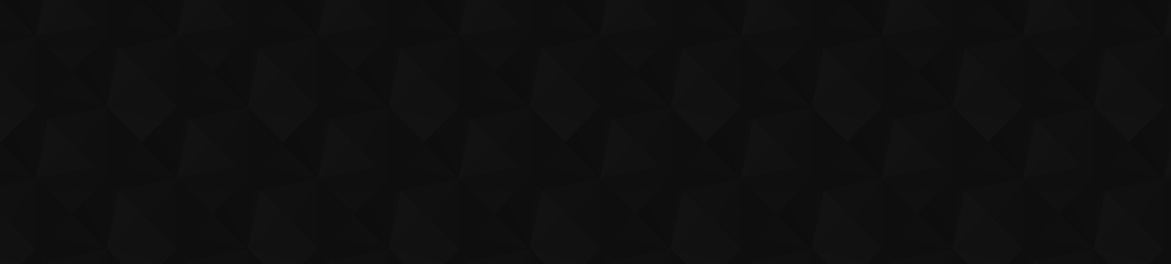
- 112
- 513 131
Stanford MLSys Seminars
United States
Registrace 11. 10. 2020
Stanford seminar on the frontier of machine learning and systems. Live every Thursday, 1:15-3 pm PT.
Pixie CEO Zain Asgar - Benefits of a Systems Mind in ML and Startups
Pixie CEO Zain Asgar - Benefits of a Systems Mind in ML and Startups
zhlédnutí: 637
Video
Pixie CEO Zain Asgar - How I Started Pixie
zhlédnutí 325Před 2 lety
Pixie CEO Zain Asgar - How I Started Pixie
Stanford PhD Albert Gu on the Research Journey behind S4
zhlédnutí 814Před 2 lety
Stanford PhD Albert Gu on the Research Journey behind S4
Stanford PhD Albert Gu Presents S4's Impressive Performance
zhlédnutí 1,1KPřed 2 lety
Stanford PhD Albert Gu Presents S4's Impressive Performance
Baharan Mirzasoleiman - How Structure Helps in Machine Learning
zhlédnutí 1,1KPřed 2 lety
Baharan Mirzasoleiman - How Structure Helps in Machine Learning
Baharan Mirzasoleiman - Dangers of Scaling Up Machine Learning
zhlédnutí 291Před 2 lety
Baharan Mirzasoleiman - Dangers of Scaling Up Machine Learning
Baharan Mirzasoleiman on Fast and Efficient Machine Learning with CRAIG
zhlédnutí 392Před 2 lety
Baharan Mirzasoleiman on Fast and Efficient Machine Learning with CRAIG
Baharan Mirzasoleiman - The Problems with Big Data in Machine Learning
zhlédnutí 511Před 2 lety
Baharan Mirzasoleiman - The Problems with Big Data in Machine Learning
Comet CEO Gideon Mendels on why industry is behind academia in machine learning
zhlédnutí 528Před 2 lety
Comet CEO Gideon Mendels on why industry is behind academia in machine learning
Comet CEO Gideon Mendels on what Tesla has to overcome for full self-driving
zhlédnutí 327Před 2 lety
Comet CEO Gideon Mendels on what Tesla has to overcome for full self-driving
Stanford MLSys Seminar Episode 0: ML + Systems
zhlédnutí 40KPřed 3 lety
Stanford MLSys Seminar Episode 0: ML Systems
I am glad you talked about inverse lithography technology (ILT), which I named twenty years ago, and I am still working on it using GPU acceleration. BTW, I also got my PhD from Stanford
... какие то откровения ML джуна
Great talk, but why didn't anyone ask questions around competition. What is to prevent Nvidia, AMD, or Intel from producing niche chips like this? With their R&D teams, Quality Assurance systems, Warranties, and supply chains, they likely thought of this and if not should be able to deploy a more competitive and reliable solution fast. That being said I really appreciate Gavin breaking down the history here I learn a lot of new things.
Corporations tend to move slowly... it is less expensive (relatively, $ and time) for a nimble co to attempt to innovate like this... also, the gamble is the Sohu platform becomes so appetizing that it ends up as an acquisition target... again, both are simply bets... not without risk
@@manonamission2000 Sure, you could argue some leaders like Blockbuster moved slow when the rising leader Netflix transitioned to online and on-demand content. However, unlike on-demand streaming services, Gen Ai is the most revolutionary technology of our time and if this direction was so promising and yet as simple as creating a niche chip focused solely on transformers then you'd think Intel and AMD with it's massive R&D teams would already be doing it to get an edge on Nvidia. These serious business questions should have been asked, I'll do more research but hard to take any of this seriously if such as basic question could not have been asked/answered.
Dude, you're at Stanford; I think students know what an inverter does. This was an ML seminar talk? How? And, how did this have anything to do with the topics explicitly raised in the Abstract? Just asking.... And, BTW, HBM isn't the only type of memory that's relevant especially for inference, which is, BTW, the focus of his company.
Awesome, I think this model will succeed one day, and transformer will lose! Remember my comment! Sheldon!
Explained so clearly! The paper gets you confused when gets into the math due to the material being so dense, thanks for extending to a video format
great presentation. thanks
This is a presentation that Ben "threw together" at the last minute? Amazingly well done!
Great talk, thanks for sharing.
What is the paper being referred to at 55:40? "Step"?
He is describing hhis company for 10 minutes apprx instead of MLOps
related to this, I'd recommend looking up the story "a disneyland without children", by strataoftheworld.
Great stuff.
Nice history lesson. Nothing about the ‘next 100x’ promised in the title.
I enjoyed the discussion and experience sharing. Thank you very much.
Amazing
Good presentation, everyone that tried this reached similar conclusions. It is great to see that confirmation and similar though process here
Thanks for the great walkthrough. Looking forward to reading these papers.
I'm not sure it makes sense to describe LLMs as lossless compressors. Wouldn't it be more accurate to say they are lossy compressors which asymptote towards becoming lossless as you train them? Ah, watched further and now see it a different way, but am still puzzled. Maybe you could anchor a different term, and say for a given level of training you can perfectly reconstruct an uncompressed message from a compressed message, and the thing that improves as training continues is the ratio of uncompressed to compressed. But then, as other commentors mention, you talk about the integral of the training loss curve. I don't get why the early and intermediate losses are relevant instead of only the end loss you can achieve. Ah, got clarification at 56:39. It makes sense to consider the integral of the loss curve only for the first epoch.
The simplest way to see an LLM as a lossless compressor is to construct an arithmetic code over the predicted probabilities. That LLMs are good at compression is not really surprising, either, it comes from the fact that there's a KL divergence embedded within the crossentropy loss used in training. (and KL(P||Q) quantifies the inefficiency of Q being used to code for P.)
danfu cooked in this one
Snippy responses 😒
Awesome work. I was looking for a resource to explain butterfly matrices and their usage and came across this talk. Invaluably helpful and an incredible contribution to deep learning.
Synthetic data generation FTW
Can we also have the slides?
Hello! Great talk! I am currently studying your SSM-related works. They are amazing! Please share your ideas, challenges, and outcomes for implementing your MAMBA model into human(sports athlete) action forecasting. Thank you for your kind reply!
This talk is more about Gemini rather than MLSys. I was expecting more on MLSys
hi, do you have any links to benchmarks you can run to measure latency, throughput for different model and frameworks etc?
Great video thanks
These videos are amazing
Stanford MLSys
37:40, as you already realized that LLM (and transformer architecture in general) is memory constrained, the extra FLOPS are wasted until TSMC productize SOT-MRAM. groq with SRAM is a more realistic short term approach for small models.
Very interesting and well presented!
Would it be possible to use an external mic for the speaker and the person who asks the question? Its quite challenging to hear
it's cool, but this is going to be a long road. The main problem is software at the IR (e.g. CUDA), not necessarily hardware. There are many companies that can make interesting transistor permutations that have been doing it for a long time and they are not magically "accelerating superintelligence". This is a software ecosystem problem more than anything else. good luck.
Great talk and even greater work!!
Ben continues to be a stud 💪💪💪 Thanks Stanford students/faculty for putting these online, they're among the beast learning opportunities for people on the sidelines 😄
(After finishing) -- What an awesome video! Data-centric modeling is awesome. Thanks MLSys for putting this on CZcams.
Ludwig da goat 🐐
Love this! Thanks!
Great presentation! Wondering if there is any literatures or papers, tutorials on the similar topics? The talk was kind of quick, need read more specifics from literatures. Any pointer would be appriciated. Thanks!
I blew through a ton of different topics in the course of the talk, so it really depends what you're looking for. If you want more on making the most of an H100, NVIDIA has fairly good docs on both the CUDA programming model as well as the specific features of the H100, but actually using them can be tricky, so your best bet is probably to read the CUTLASS repo and see how they do things. If you want more on hardware design, I'm not sure there are great alternatives to taking a class. Hardware design seems to me like an awful lot of work -- writing good RTL is hard enough, but the whole EDA stack is a bit of a nightmare. If you want more on semiconductor manufacturing, I'd highly recommend the Asianometry YT channel, which has a lot of really excellent content. Otherwise, some of my main sources for this talk were SemiAnalysis ($500/yr, but I like it enough that I pay for it even from a grad student stipend), Bill Dally's HC2023 talk, and various coursework, particularly 6.172 from MIT for performance engineering. (It's on OCW at ocw.mit.edu/courses/6-172-performance-engineering-of-software-systems-fall-2018/video_galleries/lecture-videos/ and while it's focused on CPU performance engineering many of the principles apply across both.) Hope this helps!
@@BenjaminFSpector Thanks a ton man! What you have shared here is gold. I really appreciate it.
Dan Fu == The Rizzler
Thank you for sharing this insightful video. In the introduction of Mamba, it says "parellelizable training", can you explain how parallel training is possible in an autoregressive model?
Teacher forcing
Follow this video and you will have hands on understanding why AR model could be trained in parallel. czcams.com/video/kCc8FmEb1nY/video.html
I think you might be looking for the "selective scan" part of Mamba. In section 3.3.2 of the paper arxiv.org/ftp/arxiv/papers/2312/2312.00752.pdf, they say "To avoid the sequential recurrence, we observe that despite not being linear it can still be parallelized with a work-efficient parallel scan algorithm (Blelloch 1990; Martin and Cundy 2018; Smith, Warrington, and Linderman 2023)". In short, they use a well known parallel algorithm trick to calculate a prefix sum. See en.wikipedia.org/wiki/Prefix_sum#Parallel_algorithms and you'll notice the similarity. Hope this helps!
martin is a based skyrim nord 👍😍
Wow great information I am trying to pivot my career to modelling and AI
Ben has W white boy rizz
lmao
Makes no sense, I'll ask ChatGPT for a better explanation.
The stack is a cluster-fuck, pun intended.
axriv link please?
Indeed! It is such a wonderful work and such a fantastic way to learn and I world have expected that for such a fantastic scientic exploration about this
Added to the description!
Great presentation. It is interesting to see the practical side of running a bunch of LLMs. Ops makes it happen. Coming from the old, really old, school of computing with massive multi-user, time-share systems, it is interesting to see how no matter how much computing changes, aspects of it remain the same. Through-put, latency, caching and scheduling is still central. All that seems to have changed is the problem domain. We do, in deed, live in intereswting times.
Please create a video on fine tuning MoE LLM using LoRa adapters such as Mixtural 8x7B MoE LLM within your framework