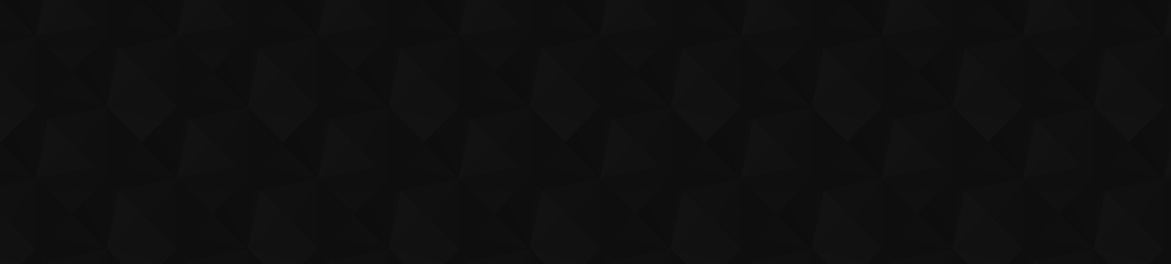
- 14
- 35 089
Yunzhu Li
United States
Registrace 3. 07. 2014
[CMU VASC Seminar] Foundation Models for Robotic Manipulation: Opportunities and Challenges
Abstract:
Foundation models, such as GPT-4 Vision, have marked significant achievements in the fields of natural language and vision, demonstrating exceptional abilities to adapt to new tasks and scenarios. However, physical interaction-such as cooking, cleaning, or caregiving-remains a frontier where foundation models and robotic systems have yet to achieve the desired level of adaptability and generalization. In this talk, I will discuss the opportunities for incorporating foundation models into classic robotic pipelines to endow robots with capabilities beyond those achievable with traditional robotic tools. The talk will focus on three key improvements in (1) task specification, (2) low-level, and (3) high-level scene modeling. The core idea behind this series of research is to introduce novel representations and integrate structural priors into robot learning systems, incorporating the commonsense knowledge learned from foundation models to achieve the best of both worlds. I will demonstrate how such integration allows robots to interpret instructions given in free-form natural language and perform few- or zero-shot generalizations for challenging manipulation tasks. Additionally, we will explore how foundation models can enable category-level generalization for free and how this can be augmented with an action-conditioned scene graph for a wide range of real-world manipulation tasks involving rigid, articulated, and nested objects (e.g., Matryoshka dolls), and deformable objects. Towards the end of the talk, I will discuss challenges that still lie ahead and potential avenues to address these challenges.
Bio:
Yunzhu Li is an Assistant Professor of Computer Science at the University of Illinois Urbana-Champaign (UIUC). Before joining UIUC, he collaborated with Fei-Fei Li and Jiajun Wu during his Postdoc at Stanford. Yunzhu earned his PhD from MIT under the guidance of Antonio Torralba and Russ Tedrake. His work stands at the intersection of robotics, computer vision, and machine learning, with the goal of helping robots perceive and interact with the physical world as dexterously and effectively as humans do. Yunzhu’s work has been recognized through the Best Systems Paper Award and the Finalist for Best Paper Award at the Conference on Robot Learning (CoRL). Yunzhu is also the recipient of the Adobe Research Fellowship and was selected as the First Place Recipient of the Ernst A. Guillemin Master’s Thesis Award in Artificial Intelligence and Decision Making at MIT. His research has been published in top journals and conferences, including Nature, NeurIPS, CVPR, and RSS, and featured by major media outlets, including CNN, BBC, The Wall Street Journal, Forbes, The Economist, and MIT Technology Review.
Homepage: yunzhuli.github.io/
Foundation models, such as GPT-4 Vision, have marked significant achievements in the fields of natural language and vision, demonstrating exceptional abilities to adapt to new tasks and scenarios. However, physical interaction-such as cooking, cleaning, or caregiving-remains a frontier where foundation models and robotic systems have yet to achieve the desired level of adaptability and generalization. In this talk, I will discuss the opportunities for incorporating foundation models into classic robotic pipelines to endow robots with capabilities beyond those achievable with traditional robotic tools. The talk will focus on three key improvements in (1) task specification, (2) low-level, and (3) high-level scene modeling. The core idea behind this series of research is to introduce novel representations and integrate structural priors into robot learning systems, incorporating the commonsense knowledge learned from foundation models to achieve the best of both worlds. I will demonstrate how such integration allows robots to interpret instructions given in free-form natural language and perform few- or zero-shot generalizations for challenging manipulation tasks. Additionally, we will explore how foundation models can enable category-level generalization for free and how this can be augmented with an action-conditioned scene graph for a wide range of real-world manipulation tasks involving rigid, articulated, and nested objects (e.g., Matryoshka dolls), and deformable objects. Towards the end of the talk, I will discuss challenges that still lie ahead and potential avenues to address these challenges.
Bio:
Yunzhu Li is an Assistant Professor of Computer Science at the University of Illinois Urbana-Champaign (UIUC). Before joining UIUC, he collaborated with Fei-Fei Li and Jiajun Wu during his Postdoc at Stanford. Yunzhu earned his PhD from MIT under the guidance of Antonio Torralba and Russ Tedrake. His work stands at the intersection of robotics, computer vision, and machine learning, with the goal of helping robots perceive and interact with the physical world as dexterously and effectively as humans do. Yunzhu’s work has been recognized through the Best Systems Paper Award and the Finalist for Best Paper Award at the Conference on Robot Learning (CoRL). Yunzhu is also the recipient of the Adobe Research Fellowship and was selected as the First Place Recipient of the Ernst A. Guillemin Master’s Thesis Award in Artificial Intelligence and Decision Making at MIT. His research has been published in top journals and conferences, including Nature, NeurIPS, CVPR, and RSS, and featured by major media outlets, including CNN, BBC, The Wall Street Journal, Forbes, The Economist, and MIT Technology Review.
Homepage: yunzhuli.github.io/
zhlédnutí: 4 793
Video
[CMU 16-831][Guest Lecture] Learning Structured World Models From and For Physical Interactions
zhlédnutí 698Před 2 měsíci
[CMU 16-831][Guest Lecture] Learning Structured World Models From and For Physical Interactions
[NeurIPS 2023] Model-Based Control with Sparse Neural Dynamics
zhlédnutí 1,6KPřed 5 měsíci
Model-Based Control with Sparse Neural Dynamics Ziang Liu, Genggeng Zhou*, Jeff He*, Tobia Marcucci, Jiajun Wu, Li Fei-Fei, and Yunzhu Li [NeurIPS 2023] robopil.github.io/Sparse-Dynamics/ (* indicate equal contribution)
[CVPR-23 Precognition] Learning Structured World Models From and For Physical Interactions
zhlédnutí 1,1KPřed 10 měsíci
Invited Talk at CVPR 2023 Workshop on Precognition: Seeing through the Future [Abstract] Humans have a strong intuitive understanding of the physical world. Through observations and interactions with the environment, we build a mental model that predicts how the world would change if we applied a specific action (i.e., intuitive physics). My research draws on insights from humans and develops m...
[PhD Thesis Defense] Learning Structured World Models From and For Physical Interactions
zhlédnutí 3,5KPřed rokem
[Abstract] Humans have a strong intuitive understanding of the physical world. We observe and interact with the environment through multiple sensory modalities and build a mental model that predicts how the world would change if we applied a specific action (i.e., intuitive physics). My research draws on insights from humans and develops model-based reinforcement learning (RL) agents that learn...
[CoRL 2021 - Oral] 3D Neural Scene Representations for Visuomotor Control
zhlédnutí 2,2KPřed 2 lety
3D Neural Scene Representations for Visuomotor Control Yunzhu Li*, Shuang Li*, Vincent Sitzmann, Pulkit Agrawal, and Antonio Torralba [CoRL 2021 - Oral] 3d-representation-learning.github.io/nerf-dy/ (* indicate equal contribution)
[IROS 2021] Dynamic Modeling of Hand-Object Interactions via Tactile Sensing
zhlédnutí 1,3KPřed 2 lety
Dynamic Modeling of Hand-Object Interactions via Tactile Sensing Qiang Zhang*, Yunzhu Li*, Yiyue Luo, Wan Shou, Michael Foshey, Junchi Yan, Joshua B. Tenenbaum, Wojciech Matusik, and Antonio Torralba [IROS 2021] phystouch.csail.mit.edu/ (* indicate equal contribution)
[ICLR-21 simDL] [Invited Talk] Compositional Dynamics Modeling for Physical Inference and Control
zhlédnutí 808Před 3 lety
Invited talk at ICLR 2021 Workshop Deep Learning for Simulation (simDL) simdl.github.io/overview/ Full Title: Learning Compositional Dynamics Models for Physical Inference and Model-Based Control
[NeurIPS 2020] Causal Discovery in Physical Systems from Videos
zhlédnutí 1,7KPřed 3 lety
Causal Discovery in Physical Systems from Videos Yunzhu Li, Antonio Torralba, Animashree Anandkumar, Dieter Fox, and Animesh Garg [NeurIPS 2020] yunzhuli.github.io/V-CDN/
[ICML 2020] Visual Grounding of Learned Physical Models
zhlédnutí 1,4KPřed 3 lety
Visual Grounding of Learned Physical Models Yunzhu Li, Toru Lin*, Kexin Yi*, Daniel M. Bear, Daniel L. K. Yamins, Jiajun Wu, Joshua B. Tenenbaum, Antonio Torralba [ICML 2020] visual-physics-grounding.csail.mit.edu/
[ICLR 2020] Learning Compositional Koopman Operators for Model-Based Control
zhlédnutí 3,3KPřed 4 lety
Learning Compositional Koopman Operators for Model-Based Control Yunzhu Li*, Hao He*, Jiajun Wu, Dina Katabi, Antonio Torralba [ICLR 2020] Spotlight Presentation koopman.csail.mit.edu/
[ICRA 2019] Propagation Networks for Model-Based Control Under Partial Observation
zhlédnutí 949Před 5 lety
Propagation Networks for Model-Based Control Under Partial Observation Yunzhu Li, Jiajun Wu, Jun-Yan Zhu, Joshua B. Tenenbaum, Antonio Torralba, and Russ Tedrake [ICRA 2019] propnet.csail.mit.edu
[ICLR 2019] Learning Particle Dynamics for Manipulating Rigid Bodies, Deformable Objects, and Fluids
zhlédnutí 10KPřed 5 lety
Learning Particle Dynamics for Manipulating Rigid Bodies, Deformable Objects, and Fluids Yunzhu Li, Jiajun Wu, Russ Tedrake, Joshua B. Tenenbaum, and Antonio Torralba [ICLR 2019] dpi.csail.mit.edu/
[NIPS 2017] InfoGAIL
zhlédnutí 1,9KPřed 5 lety
The supplementary video for our NIPS 2017 paper. InfoGAIL: Interpretable Imitation Learning from Visual Demonstrations Yunzhu Li, Jiaming Song, Stefano Ermon [NIPS 2017] Paper: arxiv.org/abs/1703.08840 Code: github.com/YunzhuLi/InfoGAIL
any links to the paper?
nice presentation. Thank you.
Good repersentation!
Excellent! I'm doing world models research and this is quite informative. Thanks Prof. Li!
Hi Li, this is very interesting work. I have a couple of questions if you may answer, 1: How do you synch the tactile and visual information? 2: Can this system predict other tasks for which it is not trained?
Hi Fahad, thank you for your interest in our work! 1. We record the timestamps for both the tactile and visual recordings. The stamps are then used to synchronize the collected frames from different data sources. 2. The test set contains motion trajectories that have different initial configurations and action sequences, but they are still from the same task that the model was trained on. We didn't test the model's generalization ability on unseen tasks, in which we would expect certain levels of generalization if the model is trained on a diversified set of tasks, but more experiments are needed to make more concrete statements.
@@yunzhuli2308 thanks.
Great work!
Inspiring!
Uber cool!
Thats brilliancy sir, what tools u used to write down the syntax and how the machines learn this exactly ? would love to know
You will be able to find more information here dpi.csail.mit.edu/, including paper and code.
thank you mr.li..appreciate what you are doin.