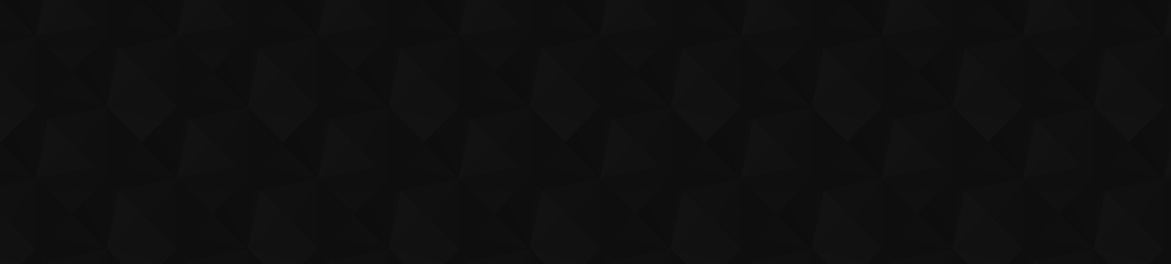
- 135
- 82 532
Machine Learning Meetups
Registrace 19. 04. 2012
Invite from Efram
Join us on next meetup at 05.03 from 17:00 in ImpactHub or watch the stream on Facebook! More information at: e/4rh8EIOn7
zhlédnutí: 14
Video
DAM: Machine learning & steelmaking industry
zhlédnutí 104Před 11 měsíci
Robert Vandlik, et al. from USS Kosice present how AI, ML and statistical modeling help in real world of steelmaking industry. In particular, following topics are discussed: - brief overview of various AI tools/models applied to different parts of steelmaking process, - more thorough description of few selected applications and their impact, - team, students and collaboration with universities.
DAIDALOS - Transhumanism in Research and in Life
zhlédnutí 162Před rokem
DAIDALOS - Transhumanism in Research and in Life The discussion "Transhumanism in Research and in Life" will explore the topic with a panel of renowned scholars, technologists and a cyborg! More info: www.aic.fel.cvut.cz/events/d-ai-dalos-transhumanism 〰️〰️〰️〰️〰️〰️ 🔴 ABSTRACT 🔴 Aside from being perceived as synonymous with cyberpunk, cryosleep and cyborgs, Transhumanism is used to seek out solu...
DAM: Machine learning - 4th pillar of space science
zhlédnutí 119Před rokem
Machine Learning is becoming the fourth pillar of scientific research. After theory, experiments, and simulations, it represents an additional essential approach to exploring nature and uncovering still hidden phenomena. Its role will be even more significant in the domain with a huge amount of data as Space Physics is. During the talk, Šimon Mackovjak will present how machine learning techniqu...
Feature Store as a Part of Modern Data Lakehouse - Online MLMU #14 - Jiří Koutný (DataSentics)
zhlédnutí 229Před 2 lety
Feature store as a part of modern data lakehouse Abstract: Over years of experience, DataSentics, as well as many other companies working with big data, begin to appreciate a structure within their data platforms. Thus, we matured from a data lake to a data lakehouse architecture and a feature store as a cherry on its top. Seemingly a minor component, the feature store covers the whole ecosyste...
MATS Stack for Machine Learning - Yury Kasimov & Joao Da Silva - Online MLMU #13
zhlédnutí 93Před 2 lety
MATS stack for machine learning Abstract: At Avast we complete over 17 million phishing detections a day, providing crucial online protection for this type of attacks. There are a lot of challenges that data scientists frequently face, such as inconsistent environments, transitioning from research to production, access management, model deployment. In this talk, Joao Da Silva and Yury Kasimov w...
Academia and Industry - Vladimir Kadlec (Seznam.cz) | MLMU.cz
zhlédnutí 120Před 3 lety
Academia and Industry - Vladimir Kadlec (Seznam.cz) | MLMU.cz
Startups do TOO MUCH RESEARCH - Martin Rehak (Resistant.AI) | MLMU.cz
zhlédnutí 255Před 3 lety
Startups do TOO MUCH RESEARCH - Martin Rehak (Resistant.AI) | MLMU.cz
Research to Value - Tomas Tunys (Rossum.AI) | MLMU.cz
zhlédnutí 100Před 3 lety
Research to Value - Tomas Tunys (Rossum.AI) | MLMU.cz
Difference between research in ACADEMIA, CORPORATE, STARTUP - Petr Marek (Promethist.AI) | MLMU.cz
zhlédnutí 89Před 3 lety
Difference between research in ACADEMIA, CORPORATE, STARTUP - Petr Marek (Promethist.AI) | MLMU.cz
NEURAL ARITHMETIC: Learning to extrapolate beyond the training range - Niklas Heim | MLMU.cz
zhlédnutí 166Před 3 lety
NEURAL ARITHMETIC: Learning to extrapolate beyond the training range - Niklas Heim | MLMU.cz
Reinforcement learning 1: deep Q networks - Michal Chovanec | MLMU
zhlédnutí 306Před 3 lety
Reinforcement learning 1: deep Q networks - Michal Chovanec | MLMU
Online MLMU #8: Sentiment Analysis for Social Media - Jan Rus
zhlédnutí 336Před 3 lety
Online MLMU #8: Sentiment Analysis for Social Media - Jan Rus
Online MLMU #3: Understanding ML with Statistical Physics - Lenka Zdeborová
zhlédnutí 1,1KPřed 4 lety
Online MLMU #3: Understanding ML with Statistical Physics - Lenka Zdeborová
Online MLMU #2: Optimization for ML: From Theory to Practice and Back - Filip Hanzely
zhlédnutí 366Před 4 lety
Online MLMU #2: Optimization for ML: From Theory to Practice and Back - Filip Hanzely
Online MLMU #1: Gestalt Principles in Data Visualisation - Anastázie Sedláková
zhlédnutí 832Před 4 lety
Online MLMU #1: Gestalt Principles in Data Visualisation - Anastázie Sedláková
Time Series Analysis in Deep Learning - Dušan Fedorčák | MLMU.cz
zhlédnutí 394Před 4 lety
Time Series Analysis in Deep Learning - Dušan Fedorčák | MLMU.cz
Image Processing in Deep Learning - Adam Kolář | MLMU.cz
zhlédnutí 159Před 4 lety
Image Processing in Deep Learning - Adam Kolář | MLMU.cz
Natural Language Processing in Deep Learning - Jiří Materna | MLMU.cz
zhlédnutí 309Před 4 lety
Natural Language Processing in Deep Learning - Jiří Materna | MLMU.cz
MLMU.cz - Marek Lipán - Applying ML to practical use-cases (Part 2)
zhlédnutí 187Před 4 lety
MLMU.cz - Marek Lipán - Applying ML to practical use-cases (Part 2)
MLMU.cz - Nikola Valešová - Applying ML to practical use-cases (Part 1)
zhlédnutí 92Před 4 lety
MLMU.cz - Nikola Valešová - Applying ML to practical use-cases (Part 1)
MLMU.cz - Strojový překlad do roku 2016 - Ondřej Bojar
zhlédnutí 151Před 4 lety
MLMU.cz - Strojový překlad do roku 2016 - Ondřej Bojar
MLMU.cz - Adam Kolář - Early Stage ML Startup, Vol.1: Research Lessons Learned
zhlédnutí 212Před 4 lety
MLMU.cz - Adam Kolář - Early Stage ML Startup, Vol.1: Research Lessons Learned
MLMU.sk: Introduction to Deep Reinforcement Learning II (Slavo Matašovský)
zhlédnutí 199Před 4 lety
MLMU.sk: Introduction to Deep Reinforcement Learning II (Slavo Matašovský)
MLMU.cz - Marek Grác - Rise of the robots: Are they ready?
zhlédnutí 127Před 4 lety
MLMU.cz - Marek Grác - Rise of the robots: Are they ready?
MLMU.sk: Introduction to Deep Reinforcement Learning (Slavo Matašovský)
zhlédnutí 178Před 4 lety
MLMU.sk: Introduction to Deep Reinforcement Learning (Slavo Matašovský)
Adopting Machine Learning Models as Part of the Business - Pablo Maldonado | MLMU.cz
zhlédnutí 123Před 4 lety
Adopting Machine Learning Models as Part of the Business - Pablo Maldonado | MLMU.cz
Deploying Machine Learning Models: Model as a Code - Boril Šopov | MLMU.cz
zhlédnutí 131Před 4 lety
Deploying Machine Learning Models: Model as a Code - Boril Šopov | MLMU.cz
Development, Deployment and Delivery of Data Products - Radovan Parrák | MLMU.cz
zhlédnutí 206Před 4 lety
Development, Deployment and Delivery of Data Products - Radovan Parrák | MLMU.cz
MLMU.sk: Save the Vineyard Hackathon - Preparatory Workshop (Stanislav Hrivňák)
zhlédnutí 34Před 4 lety
MLMU.sk: Save the Vineyard Hackathon - Preparatory Workshop (Stanislav Hrivňák)
you need to clarify the content
ρяσмσѕм
how did you do it can you share with me , thank you
The presentation starts at 6:57
Slides are here: www.slideshare.net/mobile/VaclavKosar/serve-your-ml-models-in-aws-using-python
Awesome Vid. Who's watching in 2020? Enjoy Your Day :o
Tak po roce zkoušení a trénování se mi zdá, že LSTM síť nefunguje vůbec dobře na generování věcí po písmenech a všechny ty básně, co to vygenerovalo, tak byly spíš jen náhoda a selekce. Nevěřím, že by se ta neuronová síť skutečně naučila dělat metafory. Celkem dobré výsledky jsou s transformer network, pokud člověk použije předtrénované embeddings na gigabajtech textu, ale tohle je vážné příliš jednoduchá síť a málo dat aby to byla pravda. + je zde mnohem vyšší složitost díky tomu, že to generuje po písmenech.
Neakceptovatlena kvalita zvuku :(
Don't worry, we won't eat you.
Very informative thanks....
Speaking in english language lowers the scientific value of this lecture close to zero.
Thanks, let me know what I should improve. I admit I did not prepare the text for the lecture in advance.
@@petrsvarny2880 @Petr Svarny Did you wake up from sleep after two years or what is this comment about??
@@Dr.Z.Moravcik-inventor-of-AGI I just never visited this video. Sorry for asking.
Czech language please! Prague is not in Morocco.
Use caption
Nowadays, when all idiots talk about deep learning this lecture presents little scientific value. And I wonder if all Czechs are leaving their country only to work abroad? If you are in Prague why can't you speak in your mother tongue Jan?
Tak jsem se inspiroval a teď učím LSTM síť všechny knížky Harryho Pottera, Pána Prstenů a všechny 3 díly 50 odstínů šedi naráz, schválně, jaký twist z toho vyleze. Nastavil jsem 3 LSTM vrstvy po 1536 neuronech spolu s 0.5 dropout na každé vrstvě. Generuji po písmenech a sem tam z toho vyleze smysluplná věta, ale nevím, jestli to není jenom náhodou. Příklady: "Vypadá jako ten nejlepší starý základní měsíc a madame Maxime se tvářila slunce a spatřil je jen nějakou pozornost." , "Nechci to vyslovit nic než ten zvuk. Jenže to není tak dobře, tak se na něj neochotně zamračí." , "„Pojď, Harry,“ zadržel se Harry, když vyslovil zvuk ve své podrobnosti se mnou ani nedostane do prostoru směrem. Na zadní straně do města s úsměvem a začínám se zamračí." Nedivím se, že to na básně fungovalo, protože tohle pomalu vypadá taky jako báseň. Jinak super přednáška, díky za ní!
hi, where i cat get presentation?
Very well explained! The updated presentation that OpenMined was needing
I wish that this was in English! At least, subtitles could have helped.
Hi Ishirinee. We allowed community members to add subtitles. Hopefully, someone may find time to add it.
The slide deck seems to show some interesting content being presented. It will be a shame if language becomes a barrier. Thank you.
JFYI, the game you use to illustrate a broken ladder is Lee Sedol's famous Broken Ladder game. He wound up winning the game with Black by resignation. It's commonly used as an example of a counterintuitive situation where playing out the ladder was a good thing, because it allowed Black a sente move to break the seki in the lower right. I guess this just shows that it's very difficult even for humans to evaluate who's winning ;)
Oh, it's that one! Great point. To be honest I was just rummaging through screenshots of 19x19 positions in a hurry before the talk, I didn't realize! In that case, the comment from the audience was on the spot. ;-)
For an even quicker intro, including links, see our blogpost: medium.com/rossum/building-our-own-version-of-alphago-zero-b918642bd2b5
I couldn’t find out what »PUCT« stands for at 44:49 (bottom line, »AlphaGo - PUCT«) - any ideas? Good talk, thanks in advance, cheers!
I'm actually also not terribly familiar with the PUCT algorithm, but it's described in www.ecmlpkdd2013.org/wp-content/uploads/2013/07/456.pdf - also googling "puct mcts" gets some results and code references.
Thanks for the link, Petr! That helps. PUCTS stands for »Polynomial Upper Confidence Trees«, and the paper you’ve linked to describes it in more detail than I can easily grasp. But an Upper Confidence Tree seems to be like a bunch of fused Markov chains, but for sequential, nested decisions dependent on the decisions made before. This already sounds a bit like following a board game like Go or Chess. Intriguing. Cheers!
Upper Confidence Trees are well covered in the literature and various tutorials. (They are even mentioned in the MCTS wikipedia page, though probably not in a way that's easy to understand.) I'm not sure if phrasing them as Markov chains is useful. I actually tried to explain the idea in my talk, starting at about 33:30.
Ha, yeah. I'm beginning to realize that Markov chains solve a quite different problem. I guess I’ll do what I always do: take an implementation and try to visualize how PUCTs change during usage, how they morph with time. Visualisations have always been useful to me whenever by brain wimps out reasoning about a static piece of pseudocode. :-) From sorting to differential equations to neuronal networks to any sort of nontrivial algorithm or time-changing data structure: as soon as some nice, approachable images form in my head, I can work with them much better. Happy hacking, Petr!
1:02:00 There jumps at hour 20 and hour 40 for both supervised and unsupervised version, so they're most likely external to the learning process.
Rossum? I got that reference :)
IMO, one should have L^2 neural nets, each one giving a probability of getting at least n points by the end of the game. The goal should not be to win, but to maximize points. Not all of the neural nets need to be run during play. If the neural net for 'getting AT LEAST 185 points on the board' gives a probability of e.g. 0.98, but the neural net for 'getting AT LEAST 186 points on the board' gives a probability of e.g. 0.23, Then a move should be chosen that increases the chance of getting AT LEAST 186 points while not decreasing the chance of getting AT LEAST 185 points. Assuming square board with chinese rules edge length L.
What you’re describing leads to what go players refer to as overplays. Those are bad
komi used to be only 4.5. if komi changed again, then AlphaGo would be thrown in the scrap bin, and a new one would be trained. If I played AlphaGo, and only lost by 10 points, I would be disappointed that it did not play more ruthless moves which would allow me to learn faster. The resulting program would do its best no matter what komi was chosen.
What if you had to maximize your chance of winning, but did not know what the komi is? The strategy of maximizing the number of points is sufficient to win if winning is possible.
Again, that strategy leads to overplays. The way to win is to win by the surest method. If you are ahead, you close up the shop, if you're behind you make aggressive moves and what go players refer to as "kiai" or fighting moves. With the advent of komi, the advantage has shifted to white a bit, and as such the perfect move is going to be one that assures victory in the highest number of possible continuations. That may not be the biggest move point-wise, it will be the one most guaranteed to hold on to that number of points.
I am not proposing moves that gamble, but re-prioritizing the list of valid moves. AlphaGo doesn't care about the difference between winning by 0.5 and 50 if the probability of winning is the same for both of them. We do not know what ideal komi is, the komi which would lead to a tie under perfect play. Because we do not know, we should not pretend we know, or we will produce moves that will be shown to be garbage when we finally move, because of arguments like yours. My method does not lead to overplays any more than the current AlphaZero overplays. Do you know that a Min-Max Tree search does?
For small gobans I expect playing styles of the black and of the white opponents to differ a lot. I would consider them as two different tasks and I would have designed a network having both task specific parameters and generic shared ones.
Thx for sharing
czcams.com/video/GHVaaHESrlY/video.html
Bude i záznam speakera po něm?
Hmm, teorie her je to, co dělají na ČVUT? Je čím se chlubit? Není to náhodou další pokus jak rozbít českou vědu záměrným šířením nesmyslů a hloupostí? Mne vždy učili ve škole, že život není hra. Možná i díky této dobré výchově jsem přišel na to jak lidský mozek pracuje. www.moravcik.info
Ano.. Váš mozek ale bohužel vynechává..
a kristepane 25:00 . Má toto akože byť seriózna prezentácia. "I am not sure" je asi thesis statement tejto prezentácie, je tak?
Rozsypaná prezentácia. Na ďalší raz by som si napísal, čo vlastne chcete povedať divákom.
Can you please enable subtitles so that it can reach wider Audiance.
Moc hezké, napadlo mě udělat sledovač videí na youtube, který bude přizpůsobovat rychlost přehrávání tomu, jak rychle prezentující mluví.
machine learning and cyber security
hi! where can we find the slides of this presentation?
***** I think that slides on that link is not acutal. I can send you an email with actual slides.
Jan Tkacik I would appreciate this. Do I have to add you to my google+ circles (or vice versa)?
zajimalo by me, jak funguje system trestu a odmen.. za predpokladu, ze se k vam tento komentar dostane, jak planujete odmenovat umelou inteligenci v budoucnosti.. co kdyz bez odmen nedokaze, nebo nebude chtit pracovat? hlavne byste meli rict, a nebo vedet, ze pokud vytvorite fungujici umelou inteligenci se vsim vsudy, tak ji hlavne nesmite pripojovat k internetu nebo nechavat nekde otevrene vratka, jelikoz pokud se AI rozhodne, ze lidstvo je nepodstatne a bude se nas snazit vyhubit, aby planeta prezila, tak internet je nastroj, ktereho by tim mohla dosahnout ;-)
Pěkná technická přednáška o počítačovém rozeznávání tváří. Má pro mě přesně tu správnou hloubku, protože je celá o věcech o kterých vím jen málo, ale přitom nikdy nezabředne tak hluboko, abych ztratil nit. Jsou tam části, které už jsou pro mě příliš odborné, ale jsou krátké a stačí je prostě přečkat a po minutě se zase chytím. Přitom kdybych opravdu chtěl pochopit i tyto, tak přednášející uvádí dostatek googlitelných výrazů, abych s trochou úsilí našel další informace. Také se mi líbí že uvede několik základních článků, které jsou jednotlivými milníky ve vývoji rozeznávání tváří. Doporučí i několik nástrojů, které umožňují hrát si s touto technologii zdarma. Knihovnu pro programátory, nebo demo aplikaci pro neprogramující. Na závěr i zajímavé praktické aplikace. Kdybych chodil do hospody, tak s lidmi s takovými znalostmi.
Ahoj, prosim prohodit druhou a treti prednasku v playlistu aby to bylo poporade ;) diky!