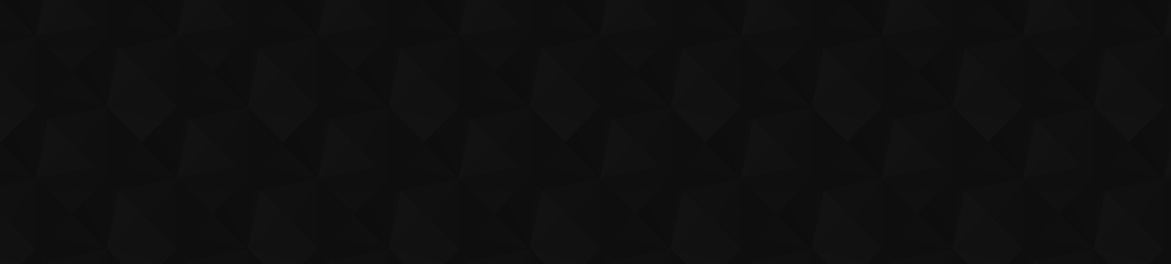
- 170
- 119 136
ML in PL
Poland
Registrace 14. 01. 2018
About us:
Founded based on the experiences in organizing of the ML in PL Conference (formerly PL in ML), the ML in PL Association is a non-profit organization devoted to fostering the machine learning community in Poland and promoting a deep understanding of ML methods. Even though ML in PL is based in Poland, it seeks to provide opportunities for international cooperation.
Founded based on the experiences in organizing of the ML in PL Conference (formerly PL in ML), the ML in PL Association is a non-profit organization devoted to fostering the machine learning community in Poland and promoting a deep understanding of ML methods. Even though ML in PL is based in Poland, it seeks to provide opportunities for international cooperation.
Katerina Stankova - Game Theory Empowered by Data Science and Machine Learning | ML in PL 23
Game Theory Empowered by Data Science and Machine Learning to Improve Treatment of Metastatic Cancer.
Standard of care in metastatic cancers typically applies Maximum Tolerable Dose (MTD) of treatment or treatment combinations, either continuously or in repeated identical cycles, until unacceptable toxicity, cancer progression, or cure. Cure is rare, due to fast evolving therapy resistance. My recent research helped to explain why MTD fails and to design first evolutionary anti-cancer therapies, i.e. therapies that anticipate and forestall evolution of treatment-induced resistance in cancer cells. Such therapies can be designed through Stackelberg evolutionary games (SEGs), i.e. games between a rational leader (here physician) and evolutionary followers (here evolving cancer cells). I will demonstrate how data science and machine learning can empower game theory to design the evolutionary anti-cancer therapies and how such therapies lead to better quality and quantity of patients’ lives.
The talk was delivered during ML in PL Conference 2023 as a part of Contributed Talks. The conference was organized by a non-profit NGO called ML in PL Association.
ML in PL Association website: mlinpl.org/
ML In PL Conference 2023 website: conference2023.mlinpl.org/
ML In PL Conference 2024 website: conference.mlinpl.org/
---
ML in PL Association was founded based on the experiences in organizing of the ML in PL Conference (formerly PL in ML), the ML in PL Association is a non-profit organization devoted to fostering the machine learning community in Poland and Europe and promoting a deep understanding of ML methods. Even though ML in PL is based in Poland, it seeks to provide opportunities for international cooperation.
Standard of care in metastatic cancers typically applies Maximum Tolerable Dose (MTD) of treatment or treatment combinations, either continuously or in repeated identical cycles, until unacceptable toxicity, cancer progression, or cure. Cure is rare, due to fast evolving therapy resistance. My recent research helped to explain why MTD fails and to design first evolutionary anti-cancer therapies, i.e. therapies that anticipate and forestall evolution of treatment-induced resistance in cancer cells. Such therapies can be designed through Stackelberg evolutionary games (SEGs), i.e. games between a rational leader (here physician) and evolutionary followers (here evolving cancer cells). I will demonstrate how data science and machine learning can empower game theory to design the evolutionary anti-cancer therapies and how such therapies lead to better quality and quantity of patients’ lives.
The talk was delivered during ML in PL Conference 2023 as a part of Contributed Talks. The conference was organized by a non-profit NGO called ML in PL Association.
ML in PL Association website: mlinpl.org/
ML In PL Conference 2023 website: conference2023.mlinpl.org/
ML In PL Conference 2024 website: conference.mlinpl.org/
---
ML in PL Association was founded based on the experiences in organizing of the ML in PL Conference (formerly PL in ML), the ML in PL Association is a non-profit organization devoted to fostering the machine learning community in Poland and Europe and promoting a deep understanding of ML methods. Even though ML in PL is based in Poland, it seeks to provide opportunities for international cooperation.
zhlédnutí: 53
Video
Volodymyr Saviak-Hewlett Packard Enterprise Tools for the AI/ML Development & Training | ML in PL 23
zhlédnutí 40Před dnem
Hewlett Packard Enterprise (HPE) offers hardware infrastructure and software tools to build, train, tune and deploy large-scale AI. In the presentation, you will learn about HPE AI at Scale software portfolio, including Machine Learning Development Environment (MLDE), Machine Learning Data Management (MLDM), Smart-SIM, Swarm Learning. More than 25 years delivers IT infrastructure solutions on C...
Błażej Osiński - Toward Efficient Machine Learning For Sequential Decision Making | ML in PL 23
zhlédnutí 53Před 14 dny
In this talk, I will present my personal journey toward developing efficient machine learning methods for sequential decision making. This journey spans both academia and industry, tackling diverse challenges that range from Atari games to autonomous driving and robotics. Why should we care about efficient methods for sequential decision making? If we observe the progress of artificial intellig...
Adam Polak - The Story Of Explainable Clustering | ML in PL 23
zhlédnutí 85Před 14 dny
This will be a talk about the problem of explainable clustering in the setting first formalized by Dasgupta, Frost, Moshkovitz, and Rashtchian (ICML 2020). A k-clustering is said to be explainable if it is given by a decision tree where each internal node splits data points with a threshold cut in a single dimension (feature), and each of the k leaves corresponds to a cluster. I will present an...
Michał Nowicki - Multi-Model Mobile Robot Localization | ML in PL 23
zhlédnutí 81Před 21 dnem
The proposed presentation concerns the new solutions for localization with multi-sensory systems, starting with the challenges of multi-sensory localization solutions from exact extrinsic calibration to non-usual sensory stacks. We will discuss camera-3D LiDAR spatiotemporal calibration through bus calibration, multi-sensory place recognition, and haptic localization. The presented solutions cr...
Gergely Neu - Importance-Weighted Offline Learning Done Right | ML in PL 23
zhlédnutí 106Před 28 dny
We study the problem of offline policy optimization in stochastic contextual bandit problems, where the goal is to learn a near-optimal policy based on a dataset of decision data collected by a suboptimal behavior policy. Rather than making any structural assumptions on the reward function, we assume access to a given policy class and aim to compete with the best comparator policy within this c...
Jiri Matas - Neural Network Deployment | ML in PL 23
zhlédnutí 80Před měsícem
Neural Network Deployment - Dealing With Non-Differentiable Objectives and With Prior Shift The talk will touch two problems that are common, yet rarely addressed, in the deployment of system based on neural networks. The natural formulations of many tasks, in computer vision and elsewhere, leads to a non-differentiable objective function, rendering standard SGD training inapplicable. In applic...
Sneha Jha - Memory Optimization for Finetuning Models | ML in PL 23
zhlédnutí 121Před měsícem
Large deep learning models often require memory far in excess of processing units typically available. This presents unique challenges in fine-tuning models with a large number of parameters. We aim to discuss the group of memory optimization techniques such as low-rank adaptation, quantization etc applicable to models like the recent LLMs. These techniques are scattered across the literature a...
ML in PL Conference 2023 Aftermovie [short version]
zhlédnutí 179Před měsícem
While waiting for the next opportunity to meet, let's remember the highlights of the ML in PL Conference 2023! Make sure to follow us on social media to not miss any news about ML in PL Conference 2024: 👉 ML in PL LinkedIn Page: www.linkedin.com/company/mlinpl/ 👉 ML in PL Facebook Page: MLinPL 👉 ML in PL Twitter Profile: MLinPL 👉 ML in PL Conference Website: con...
Charles O'Neill - Steering Language Generation | ML in PL 23
zhlédnutí 71Před měsícem
Steering Language Generation: Harnessing Contrastive Guidance and Negative Prompting for Coherent and Diverse Synthetic Data Generation Large Language Models (LLMs) hold immense potential to generate synthetic data of high quality and utility, which has numerous applications from downstream model training to practical data utilisation. However, contemporary models, despite their impressive capa...
Piotr Rybak - The State Of Polish Semantic Search In The Era Of LLMs | ML in PL 23
zhlédnutí 150Před měsícem
As Large Language Models (LLMs) gain popularity, concerns about hallucination are becoming more prevalent. One effective approach to addressing this issue is to augment the prompt with related documents, allowing LLMs to rely on existing information rather than generating facts. However, the challenge is to identify relevant documents efficiently and accurately. In this talk, I will give an ove...
Paulina Tomaszewska - Deep Spatial Context - Digital Pathology | ML in PL 23
zhlédnutí 99Před měsícem
Neural networks find application in the processing of histopathological images. However, it comes with some challenges. One of the most popular approaches is an attention-based model. In the talk, the Deep Spatial Context method will be presented, which was inspired by the need to analyse whether the behaviour of the trained models aligns with the histopathologists' good practices that take int...
Maciej Wiatrak - Fighting Antibiotic Resistance With Deep Learning | ML in PL 23
zhlédnutí 71Před měsícem
Rapid diagnosis of antibiotic-resistant bacteria and understanding the molecular mechanisms of Antimicrobial resistance (AMR) is a major unsolved problem which poses a significant threat to global public health. Here, we report substantially improved antibiotic resistance prediction from DNA sequences through the introduction of a novel deep learning architecture called GeneBac. We show that by...
Maciej Wysocki-The Future Is Now: Embracing Allegro Transactional Data For Risk Modeling|ML in PL 23
zhlédnutí 100Před měsícem
The accurate prediction of probability of default plays a critical role in safeguarding Allegro Pay business. Industry standard models, like tree-based models, unfortunately fall short in capturing the complexities of time-varying transactional data, limiting their predictive capabilities in prescoring applications. This presentation unveils a groundbreaking approach that harnesses the power of...
Bartosz Ptak - Enhancing Lunar Robotics Rover Through Deep Learning And Edge AI | ML in PL 23
zhlédnutí 82Před měsícem
With the growing interest in space exploration, challenges are also increasing. Recent years have witnessed a notable surge in the advancement of space robotics, particularly pertaining to Moon exploration. The incorporation of Commercial off-the-shelf (COTS) devices in this domain has expedited both research and development endeavours. It also enables equipping satellites, rovers and landers i...
Philippe Preux - Bits of Reinforcement Learning | ML in PL 23
zhlédnutí 126Před 2 měsíci
Philippe Preux - Bits of Reinforcement Learning | ML in PL 23
Piotr Płoński - Create user interface for machine learning models directly from python notebook
zhlédnutí 281Před 3 měsíci
Piotr Płoński - Create user interface for machine learning models directly from python notebook
Maja Jabłońska-Unlocking scientific discoveries with large language models in astronomy|ML in PL 23
zhlédnutí 116Před 3 měsíci
Maja Jabłońska-Unlocking scientific discoveries with large language models in astronomy|ML in PL 23
Piotr Januszewski - Mastering MLops at a reasonable scale at allegro | ML in PL 23
zhlédnutí 80Před 3 měsíci
Piotr Januszewski - Mastering MLops at a reasonable scale at allegro | ML in PL 23
Jane Dwivedi Yu - Teaching Language models to use tools | ML in PL 23
zhlédnutí 305Před 3 měsíci
Jane Dwivedi Yu - Teaching Language models to use tools | ML in PL 23
Grzegorz Jacenków-Privacy distillation:reducing reidentification risk of multimodal diffusion models
zhlédnutí 54Před 3 měsíci
Grzegorz Jacenków-Privacy distillation:reducing reidentification risk of multimodal diffusion models
Kamil Deja - Diffusion models - what we already can and yet can't generate | ML in PL 23
zhlédnutí 394Před 3 měsíci
Kamil Deja - Diffusion models - what we already can and yet can't generate | ML in PL 23
Michał Jamroż - Class fitting in residual convolutional networks | ML in PL 23
zhlédnutí 71Před 4 měsíci
Michał Jamroż - Class fitting in residual convolutional networks | ML in PL 23
Sebastian Cygert - Toward continually learning models | ML in PL 23
zhlédnutí 133Před 4 měsíci
Sebastian Cygert - Toward continually learning models | ML in PL 23
Desmond Elliot - Language modelling with pixels | ML in PL 23
zhlédnutí 197Před 4 měsíci
Desmond Elliot - Language modelling with pixels | ML in PL 23
Jan Dubiński - Bucks for buckets (b4b): active defenses against stealing encoders | ML in PL 23
zhlédnutí 78Před 4 měsíci
Jan Dubiński - Bucks for buckets (b4b): active defenses against stealing encoders | ML in PL 23
Adam Dziedzic - Privacy for large language models | ML in PL 23
zhlédnutí 141Před 4 měsíci
Adam Dziedzic - Privacy for large language models | ML in PL 23
Leon Bottou - Borges and AI | ML in PL 23
zhlédnutí 1,3KPřed 4 měsíci
Leon Bottou - Borges and AI | ML in PL 23
Sayak Ray Chowdhury - Differential Privacy in Reinforcement Learning | MLSS Kraków 2023
zhlédnutí 133Před 7 měsíci
Sayak Ray Chowdhury - Differential Privacy in Reinforcement Learning | MLSS Kraków 2023
Bartosz Zieliński - Interpretable Deep Learning with Prototypical Parts | MLSS Kraków 2023
zhlédnutí 278Před 7 měsíci
Bartosz Zieliński - Interpretable Deep Learning with Prototypical Parts | MLSS Kraków 2023
Thanks
Insightful
I really love his arguments and his thought process. I wouldn't like or use an AI if it was a cold logic device. Everything that represents a human way would be lost. I mean this for a chat machine. Control machines in industry have different preferences.
Loved the talk. Thanks for sharing!
Great talk!!
Where can I find the code to do the same?
why, at 19:15, is the visualization of the slop curved? the model seems to be a degree 1 polynomial
oh its log(ax+b) i guess
This lecture is worth watching more than once
Im a young datascientist unsatisfied with my current level of knowledge. Geometric deep learning has been on my reading list for a year now. Thanks for the video, this is golden
make sure you learn your math background well, it will make the learning easier.
@@eliasmai6170which book would you recommend for this topic
Hmm I am learning data science and data analysis after dabbling with Haskell/Lispell for a bit have a degree in computer science also learning Chinese and Japanese. I am wondering if there is any way to automate social networking (Reddit, LinkedIn, CZcams, Upwork, Github, etc...) sort of like chatGPT automates writing code (i.e: you give it a query and it uses all SNS websites at once to find a result).
such an excellent talk! as the demos / alchemy era is ending and the Grassmanian era dawns 🦆
przebrnąłem przez konferencję, ogólnie nie pojmuje kilku spraw. Jestem w Polsce i muszę oglądać i słuchać Polaków męczących się po angielsku...... Gdzie tak jest na świecie? Temat jest z dotacji więc może by było warto robić coś po polsku- polski LLM też zrobimy po angielsku. Co do wykładów, poziom średni. Czemu nie zaprosicie takiego Andrieja Karpathy co opowie o aktualnych trendach, badaniach i pomysłach na architektury i skalowanie sieci neuronowych ? Czemu nie zaprosicie takiego Sebastiana Raschki, który opowie jak dostroić model, sqwantyzować, uruchomić ect. Świat ucieka do przodu a my dalej stroimy architekturę BERTA :), która nawet nie jest w stanie uczyć się z kontekstu.... lub powiększamy kontekst w Llama za unijną kasę. Świat ma laby zaopatrzone w tysiące GPU, a nasze ośrodki badawcze mają klastry obliczeniowe złożone z ... 8 sztuk A100 i to nie konieczne w architekturze DGX.. Dlatego jesteście w IV setce uczelni mając średnio jednego pracownika na aż 7,4studentów :)
The camera didn't need to pan to every questionaire, rather keeping it focused on the slide. very unsettling.
How about the other way, The Occam's razor ?
Cool lecture, but the volume is really low.
Naming conflict: pyro was Python Remote Objects 10 years ago...
Naming conflict #2: H0 is also used to represent the null hypothesis
'PromoSM'
Where is Polish translator xD
12:05 😂😂😂😂
Probabilistic Programming is same as Bayesian Statistics?
@Hobbesian Thinker Thank you!
Apparently ChatGPT has been programmed to think it's acceptable to mutilate children genitals but it's not appropriate to disagree with this.
but what is performance of Explainable Boosting Machine vs xgboost - better do not ask this question...
I have watched SO many videos on attention with lots of graphics, animations, but you explain it the best.
This is a copy of Thore Graepel talk and even slides :-(
This is a copy of Thore Graepel talk and even slides :-(
Really well explained. Going to give this a try myself. I think this is going to help our company produce safer and more responsible models.
Thanks for sharing this. Very interesting!
Wspaniala
𝙥𝙧𝙤𝙢𝙤𝙨𝙢
Such a great and inspirational talk! Thanks a lot for posting! Does anyone know whether the slides are available for download somewhere?
man of culture :D
3:44 - Now I know what you mean.
The loss function is really suspicious, and the output goes to very blurry sometimes
35:21 the formula for the posterior is wrong, isn't it? It should be p(theta|y,d) instead of p(y,theta|d).
I think that's just simpe formulation of conditional probability, the starting formular of Bayes' Theorem first equation in en.wikipedia.org/wiki/Conditional_probability
Possible to share the source code for this?
Super underrated lecture it deserves much more views! Great explanation thank you!
Fajne wystąpienie! Greetings!
Can somebody please let me know where can I get the literature for this talk?
Machine learning is dead . Long live AI
Great talk, thanks for uploading
Sir can you please provide the dataset of this images.
Hi have you got any pre-processed datasets for breast cancer classification ?
@@pranabsarma18 yes I have ping me on my email harshit.shakya99@gmail.com
Please advise me a best dataset for breast cancer preprocessing and classification. liaqattops@gmail.com
can we get the slides from somewhere?
Can you make a tutorial about how to reconstruct missing parts of a damaged video?
Hi stranger, you can find some jupyter notebooks with read-to-use code if you type in google "inpainting auto encoder pytorch site:github.com", or replace pytorch with tensorflow if you prefer
@@robke136 this is a good suggestion
Thanks
Can I have this presentation?
Nice, very practical talk with lots of idea combined
Good stuff guys. Keep up such work and ideas 👍
Thank you for this digest of information! Intuitions and mini-summaries are a real goldmine
Rybak... ten łajdacki prowokator?
can ı have the presentation?
Wondering if the slides are posted anywhere? Thanks!
www.cs.cmu.edu/~rsalakhu/