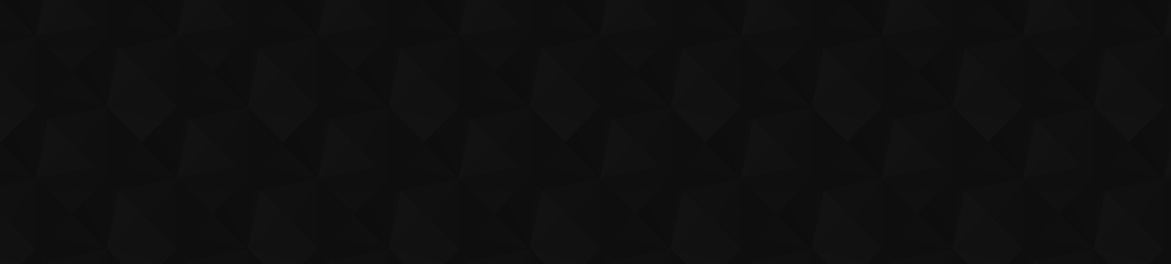
- 19
- 95 970
Jaejun Yoo
Registrace 10. 10. 2010
PR-400: Score-based Generative Modeling Through Stochastic Differential Equations
- Jaejun Yoo
(Korean) Introduction to Score-based Generative Modeling Through Stochastic Differential Equations (ICLR 2021)
Paper: openreview.net/forum?id=PxTIG12RRHS
(Korean) Introduction to Score-based Generative Modeling Through Stochastic Differential Equations (ICLR 2021)
Paper: openreview.net/forum?id=PxTIG12RRHS
zhlédnutí: 9 632
Video
PR-385: Generative Modeling by Estimating Gradients of the Data Distribution
zhlédnutí 5KPřed 2 lety
- Jaejun Yoo (Korean) Introduction to Generative Modeling by Estimating Gradients of the Data Distribution (NeurIPS 19 Oral) Paper: arxiv.org/abs/1907.05600
PR-374: Fourier Features Let Networks Learn High-Frequency Functions in Low Dimensional Domains
zhlédnutí 3,8KPřed 2 lety
(Korean) Introduction to Fourier Features Let Networks Learn High Frequency Functions in Low Dimensional Domains Paper: arxiv.org/abs/2006.10739
PR-363: Sketch Your Own GAN
zhlédnutí 664Před 2 lety
(Korean) Introduction to Sketch Your Own GAN Paper: arxiv.org/abs/2108.02774
PR-349: Adversarial Generation of Continuous Images
zhlédnutí 854Před 2 lety
(Korean) Introduction to Adversarial Generation of Continuous Images Paper: arxiv.org/abs/2011.12026
PR-338: Alias-Free Generative Adversarial Networks (StyleGAN3)
zhlédnutí 4KPřed 2 lety
(Korean) Introduction to Alias-Free Generative Adversarial Networks (StyleGAN3) Paper: arxiv.org/abs/2106.12423
PR-323: Separation and Concentration in Deep Networks
zhlédnutí 1KPřed 3 lety
(Korean) Introduction to Separation and Concentration in Deep Networks Paper: openreview.net/forum?id=8HhkbjrWLdE
PR-312: Generative Models as Distributions of Functions
zhlédnutí 2,3KPřed 3 lety
(Korean) Introduction to Generative Models as Distributions of Functions Slide: www.slideshare.net/thinkingfactory/pr12-generative-models-as-distributions-of-functions Paper: arxiv.org/abs/2102.04776
[CVPR 2020] Simple but Effective Image Enhancement Techniques
zhlédnutí 4,4KPřed 4 lety
CVPR company session, NAVER, Clova AI. I gave a talk in the company session on behalf of Clova AI research team. These are the recent projects that I did from 2019 and 2020 when I was in NAVER. (ICCV'19, CVPR'20, CVPRW'20) The slide link is at: www.slideshare.net/thinkingfactory/cvpr2020-simple-but-effective-image-enhancement-techniques
Rethinking Data Augmentation for Image Super-resolution: A Comprehensive Analysis and a New Strategy
zhlédnutí 1KPřed 4 lety
CVPR 2020 Paper: arxiv.org/abs/2004.00448 Github: github.com/clovaai/cutblur Slides: www.slideshare.net/thinkingfactory/rethinking-data-augmentation-for-image-superresolution-a-comprehensive-analysis-and-a-new-strategy Twitter: bit.ly/2SDj35c Abstract: Data augmentation is an effective way to improve the performance of deep networks. Unfortunately, current methods are mostly developed for high-...
PR-087: Spectral Normalization for Generative Adversarial Networks
zhlédnutí 6KPřed 6 lety
(Korean) Introduction to Spectral Normalization for Generative Adversarial Networks (ICLR 2018 Oral) Slide: www.slideshare.net/thinkingfactory/pr12-spectral-normalization-for-generative-adversarial-networks Paper: openreview.net/forum?id=B1QRgziT-
PR-071: Categorical Reparameterization with Gumbel Softmax
zhlédnutí 7KPřed 6 lety
(Korean) Introduction to (paper1) Categorical Reparameterization with Gumbel Softmax and (paper2) The Concrete Distribution: A Continuous Relaxation of Discrete Random Variables Slide: www.slideshare.net/thinkingfactory/pr12-categorical-reparameterization-with-gumbel-softmax Paper1: arxiv.org/abs/1611.01144 Paper2: arxiv.org/abs/1611.00712
PR-061: Understanding Deep Learning Requires Rethinking Generalization
zhlédnutí 4,1KPřed 6 lety
Introduction to the ICLR best paper " Understanding Deep Learning Requires Rethinking Generalization" (KOR) Slides: www.slideshare.net/thinkingfactory/pr12-understanding-deep-learning-requires-rethinking-generalization
PR-056: Capsule Network
zhlédnutí 6KPřed 6 lety
Introduction to CapsNet (or Capsule Network) Slides: www.slideshare.net/thinkingfactory/pr12-capsule-networks-jaejun-yoo Paper: Dynamic Routing Between Capsules
PR-013: Domain Adversarial Training of Neural Network
zhlédnutí 8KPřed 7 lety
PR-013: Domain Adversarial Training of Neural Network
6년 전 자료라는게 놀랍네요. 설명뿐 아니라 질문들 또한 너무 좋았습니다.
Could you kindly provide the pseudocode?
fourier feature가 어떻게 high frequency 들에 잘 피팅되게 하는건가요? 그니까음 ntk 가 stationary 해지는 것과 high freq에 잘 피팅된다는 것 과 어떻게 연관이 있는 건가요???
its cool sir
super resolution에서 좋겠네요 잘 봤습니다
5:00 likelihood 가 tracktable 하다는게 아직 이해가 안가네요 (한번 더 영상보고 답글에 답 달아보겠습니다..!)
z 가 intracktable 한 이유는 모든 z 를 추적할 수 없기 때문인데 모든 p(x | p x_i) 는 추적할 수 있는걸까요
그렇다. P(X) 를 알아낼때 marginal prob 를 알아내는 것은 불가능하지만 chain prob 곱을 알아내는 건 가능한 것이다.
if this video was in english :)
감사합니다~!
교수님, Consistency Model 분석 해주시면 안될까요?
wow, though I can't understand what you are talking, still getting some useful information from your nicely done ppt
덕분에 아웃라인을 잡고 논문 읽는데 도움이 많이 되었습니다! 감사합니다
1. sampling 은 미분이 안됨. 2가지 remedy 가 제시됨 - 외주 (reparameterization trick) : stochastic 한 부분은 업데이트가 일어나지 않는 분포에서 random 값 가져와서 업데이트가 일어나는 discrete한 부분과 더해줌 - 치환 (score function estimator) : 확률 형태를 뽑기 위해 d logx = 1/x 의 성질 이용해서 식을 바꿈. 2. 1의 방법들은 continuous 한 z 를 뽑을 때는 좋은데 discrete 한 z 를 뽑을 때는 적용하기 곤란함 - 미분가능한 discrete variable 샘플링 방법인 gumbel softmax 도입. - 외주 방법(gumbel noise) 을 통해 랜덤성을 확보하고, temperature + softmax 를 통해 argmax 역할을 대체하면서 미분도 가능함
안녕하세요, 유교수님 김민규입니다. 요새 INRs 관련 연구들을 팔로우업 하고 있는데 너무 유익한 세미나 해주셔서 감사합니다~ 이에 더해 NTK를 이렇게 자연스럽게 설명해주실 수 있는 분도 어디있을까싶네요! 또 이렇게 도움받아 갑니다~
감사합니다 유재준 교수님! 어려운 논문 너무 이해하기 쉽게 설명해주셔서.... ㅠ
32:49 "구지 깔끔한 x 에 noise를 넣어주지?" 제 소견으로는 y대신 깔끔한 x를 사용하면 또 다른 의미에서 low density 영역을 사용하게 되기 때문에 좋지 않은 방법인 것 같습니다. 반쪽은 깨끗한 관측 데이터이고 반쪽은 noise로 채워진 데이터는 스코어 함수 학습시에 보지 못했을 것입니다. 이런 x를 그대로 사용하는 것 보다는 signal은 x에서 가져오되 전체적인 noise의 비율은 스코어 함수를 학습할 때와 최대한 비슷한 형태가 되도록 y를 만들어 사용하는 것이 보다 좋은 성능을 기대할 수 있는 자연스러운 방법이지 않나 생각이 듭니다.
What's the point of having a title in english if there are no subtitles?
course name being english, its common for other language classes
FR
Talk about stating the bleeding obvious.
me
굉장한 설명 감사합니다. 25:50 논문 구현에서 f(x,t)는 구체적으로 무엇인가요? ddpm에서 sqrt(1-beta)*x_t의 극한 모양일것 같다고 예상됩니다만.
czcams.com/video/d_x92vpIWFM/video.html 이 f(x,t) and g(t)의 모양이 DDPM에서 어떻게 되는건지 설명합니다. 그걸 이해하고 나니 30:00 에서 무슨 이야기를 하는지도 이해가 되네요.
와 정말 이해가 잘되는 강의였습니다! 다른분들 질문도 그렇고 대답하시는 강연자분도 그렇고 이해에 도움이 되는 질의가 가득하네요
잘 설명해주셔서 감사합니다! 교수님 덕분에 새로운 인사이트를 얻고 갑니다!!
듣고 나니 감동의 눈물이 흘러내렸습니다 교수님 감사합니다...
11:30 DDPM은 clever하게 ∆ logP == N(0,1)이 되는 상황을 만들어 그냥 fisher divergence를 loss로 쓴거였군요.
좋은 강의 감사합니다~
유익한 영상 감사합니다! 논문 읽으면서 NTK 에 대해서도 어렴풋이 이해하고 있었는데 알기 쉽게 설명해주셔서 너무 좋네요 ㅎㅎ
도움이 되셨다니 다행입니다.
미친 설명입니다! 'Lil'Log: What are Diffusion Models?' 블로그 읽고 'score matching이 뭐지?' 하고 넘어갔었는데, 잘 배웠습니다.
대충만 알고 있었는데 듣고 나니 전체적으로 연결이 되네요 ㅎ 감사합니다 ^^
최강설명...
문득 든 생각인데, 만약에 입력이 좌표가 아니라 이미지나 음성이라면, 입력 신호의 주파수를 변조해서 뉴럴넷의 입력으로 넣으면 신호를 복원할 때 효과가 있을까요? ㅎㅎ
그렇게 하도록 한게 Fourier feature라고 생각하고 있긴 합니다 ㅎㅎ
여윽시 유교수님 ㅋㅋ 재밌는 얘기들 많이 듣고 갑니다 ㅎㅎ
ㅎㅎ 감사합니다 광민님 잘 지내시죠? ㅎㅎ
@@jaejunyoo 넵 그럭저럭요 ㅎㅎ 다시 학생으로 돌아간다면 유교수님 학생이 되고싶군요 ㅋㅋ 종종 재밌는 얘기들 들려주세요 ㅎ 이번 리뷰도 유익했네요 ^^
너무너무 좋은 자료 감사드립니다
이해하는데 많은 도움이 되었습니다. 감사합니다. 😀
Hi, can you share the presentation?
감사함니다
감사합니다
Please upload it in english .
좋은 강의 감사합니다!!
확실히 cnn보다 미래지향적인 방향으로 보입니다!
Implicit neural Representation에 대한 의문이 확 풀렸습니다 감사합니다~
도움이 되셨다니 다행입니다.
유재준 교수님 :D 오늘도 많이 배우고 갑니다 !!!
요섭형!!!!! ㅋㅋ 보고싶습니다.
@@jaejunyoo 아이고 ㅋㅋ 우리 유재준 교수님 ㅋㅋㅋ LAIT 에 무궁한 발전을 기원합니다 !! 연구실이 너무 멋집니다 😄 부러웡 ㅋㅋㅋ
감사합니다. :-) 설명 잘 들었습니다.
감사합니다. 상당히 흥미롭군요
흥미로운 논문 잘 배웠습니다. 내용의 깊이와 넓이, 전달력 모두 최고시네요. 최근에 MLP-Mixer에서 MLP를 FFT로 교체한 FNet이라고 나왔는데, FFT를 scatter layer로 교체해서 누가 Scatterfomer논문 쓰면 될것 같습니다. czcams.com/video/JJR3pBl78zw/video.html
잘봤습니다. 좋은영상 감사합니다
35페이지짜리 논문을 35분만에 볼 수 있다니..!
we need AI to translate this into english...
설명을 왜 이렇게 잘하세요
좋은 설명 감사합니다. 그런데 혼동되는 부분이 있네요.. Transform Matrix 들은 Training 대상이고, cij (capsule-wise scale factor) 는 inference 과정 중 feature에 따라 dynamic 하게 계산되어야 하는 것이지요?
설명 정말 잘 들었습니다. 이미지 인식 모델에 한번 적용해 봐야겠어요 :)
Sir can you plz translate it in English ..
제가 깨달은 바를 바탕으로 15:08 Diagonal biLSTM 파트 부연설명하자면, 오른쪽->왼쪽 hidden state들을 왼쪽->오른쪽 hidden state에 간편하게 더해주기 위해서, 15:15와 같은 방식으로 parallelization 하되(이 경우 왼쪽으로 skew하겠죠) 아웃풋 맵을 전부 한칸씩 밑으로 내려서, 왼쪽->오른쪽 hidden state에 더합니다. 한칸 씩 밑으로 안 내린다면 해당 픽셀이 미래 정보(자기와 같은 열 오른쪽에 있는)를 보게됩니다.