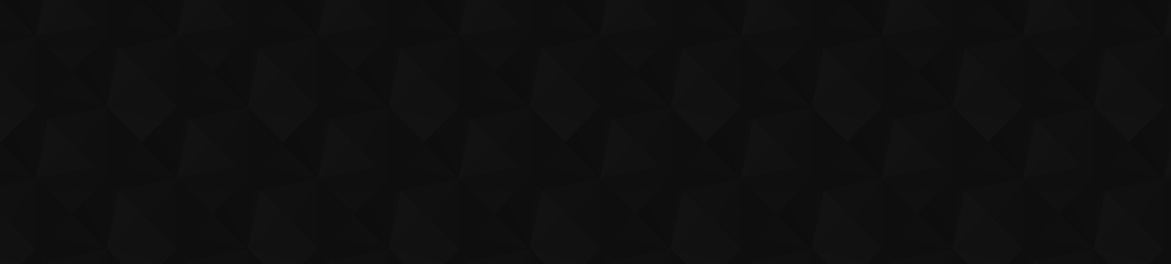
- 6
- 5 671
Let's Learn Transformers Together
Registrace 9. 02. 2016
Transformer Encoder vs LSTM Comparison for Simple Sequence (Protein) Classification Problem
The purpose of this video is to highlight results comparing a single Transformer Encoder layer to a single LSTM layer for a very simple problem. Several texts on Natural Language Processing describe the power of LSTM as well as the advanced sequence processing capabilities of Self Attention and the Transformer. This video offers very simple results in support of these notions in the field of Natural Language Processing.
Previous Video:
czcams.com/video/9V4xgt3Vs8A/video.html
Code:
github.com/BrandenKeck/pytorch_fun
Interesting Post:
ai.stackexchange.com/questions/20075/why-does-the-transformer-do-better-than-rnn-and-lstm-in-long-range-context-depen
Music Credits:
Breakfast in Paris by Alex-Productions | onsound.eu/
Music promoted by www.free-stock-music.com
Creative Commons / Attribution 3.0 Unported License (CC BY 3.0)
creativecommons.org/licenses/by/3.0/deed.en_US
Small Town Girl by | e s c p | www.escp.space
escp-music.bandcamp.com
Previous Video:
czcams.com/video/9V4xgt3Vs8A/video.html
Code:
github.com/BrandenKeck/pytorch_fun
Interesting Post:
ai.stackexchange.com/questions/20075/why-does-the-transformer-do-better-than-rnn-and-lstm-in-long-range-context-depen
Music Credits:
Breakfast in Paris by Alex-Productions | onsound.eu/
Music promoted by www.free-stock-music.com
Creative Commons / Attribution 3.0 Unported License (CC BY 3.0)
creativecommons.org/licenses/by/3.0/deed.en_US
Small Town Girl by | e s c p | www.escp.space
escp-music.bandcamp.com
zhlédnutí: 195
Video
A Very Simple Transformer Encoder for Protein Classification in PyTorch
zhlédnutí 183Před měsícem
The purpose of this video is apply previously explored transformer encoder approaches to protein language learning and large multiclass classification problems using the protein family (PFam) dataset. Code Repo: github.com/BrandenKeck/pytorc... Attention Is All You Need: arxiv.org/pdf/1706.03762.pdf Music Credits: Eternal Springtime by | e s c p | www.escp.space escp-music.bandcamp.com Gate by ...
Conv1D for Embedding Timeseries for Forecasting with Transformers
zhlédnutí 392Před měsícem
EDIT: As an additional note, Conv1D layers are good for sequence analysis in general. I had never thought of them as an "embedding" layer, but from this perspective it feels very natural. The purpose of this video is to highlight something that I learned after reading comments on my last video: Conv1D embedding is possibly a preferable option to Linear embedding for timeseries because it can le...
A Very Simple Transformer Encoder for Time Series Forecasting in PyTorch
zhlédnutí 3,6KPřed 2 měsíci
The purpose of this video is to dissect and learn about the Attention Is All You Need transformer model by using bare-bones PyTorch classes to forecast time series data. Code Repo: github.com/BrandenKeck/pytorch_fun Very helpful: github.com/oliverguhr/transformer-time-series-prediction/blob/master/transformer-singlestep.py github.com/ctxj/Time-Series-Transformer-Pytorch github.com/huggingface/t...
Transformer Attention for Time Series - Follow-Up with Real World Data
zhlédnutí 466Před 2 měsíci
In a previous video (czcams.com/video/k23iXPyJ-as/video.html) I looked at an approach to using Transformer Attention in time series forecasting. The data used to test the model in that video was extremely simple. In this video, the model is tested against more complicated data and some implications of the model are discussed. Code: github.com/BrandenKeck/pytorch_fun Attention Is All You Need: a...
Transformer Attention (Attention is All You Need) Applied to Time Series
zhlédnutí 846Před 2 měsíci
The purpose of this video is to highlight a very basic implementation of Attention to time series. This was a problem of interest that I struggled with. Hopefully this video helps anyone else who has interest in this problem. As mentioned in the video, here is a link to the code: github.com/BrandenKeck/pytorch_fun Attention Is All You Need: arxiv.org/pdf/1706.03762.pdf I've noticed that the cod...
Zamm!
I never knew that transformers were that much more time efficient at large embedding sizes
Hey @LeoDaLionEdits - I'm very interested in ideas like these. I unfortunately lost my link to the paper - but there was an interesting arXiv article on why XGBoost still dominates Kaggle competitions in comparison to Deep Neural Networks. Based on the problem, I think RNN / LSTM may often be more competitive in the same way: the simpler, tried-and-true model winning out. From a performance perspective, this book notes the advantage in parallel processing of transformers in sections 10.1 (intro) and 10.1.4 (parallelizing self-attention): web.stanford.edu/~jurafsky/slp3/ed3book.pdf
Thanks!
Thank you for your knowledge sharing. Can the code run on the jupyter notebook as well?
Thanks @elmojuanara5628! The code should run just fine in a notebook - some additional work may be required based on GPU availability of the notebook, but I believe some services such as Colab handle this very well for CUDA.
Please....... make more videos on this paper and also transformed time series
Thank you @alihajikaram8004! I am in the process of studying some applications to Protein/Molecule data, however I'd like to explore some more advanced approaches for timeseries soon!
@@lets_learn_transformers I can't wait to see more videos from you (especially about time series)
Love the video's and your presentation style!
Thank you!
Thank you!
Pls put more videos you are awesome ❤❤❤ good luck 🙏🏻
Thank you!
Does using Conv1D to generate input embeddings improve your output predictions?
Hi @rdavidrd, I did not observe an improvement in the limited testing I did. However, the problems used here are very basic and I did not do any rigorous tuning to improve the models. I left results out of this video for this reason - because I didn't want to make any statements on Conv1D being better without specific results. My intuition is that Conv1D is an improvement, but I believe this is problem-specific and would require some experimentation. Sorry for a bit of a non-answer, but I hope this helps!
@@lets_learn_transformers No need to apologize-your response is informative and highlights important considerations for others exploring similar methods. Thanks for your input! Maybe using LSTMs instead of Conv1D (or using both) could be an avenue worth exploring.
Great content. Any intention to illustrate a multivariate time series model? I am doing experiments on this, using each time step (of x features) as a ‘token’ and embedding it using a Linear layer (x, embed_size). I am wondering if there are better ideas for this.
Thanks @naifaladwani9181! I do not have plans to illustrate a multivariate time series, as I plan on shifting topics for a few videos. However, you could also use the Conv1D layer in this case - if you replace the first argument in nn.Conv1D (in_channels) with the size of the data at each time step, the output dimensions should be the same (I will have to double check this)
When you say you extended the forecasting window, do you mean that the model now outputs more time step predictions or are you still just predicting one timestep into the future and unrolling the model for more days?
Hi @isakwangensteen6577 - sorry for the lack of clarity. I mean that the model now outputs more time step predictions!
Pls keep going do more videos
Thanks!
Hey great content, please keep educating
Thank you!
Thank you for the mention and for the clear video ! I still have questions (I am running experiments on them) regarding the optimal size of tokens (pointwise vs sub sequence wise). Also, what to do when you have multiples features / multivariate time series.
Thanks @jeanlannes! This is very interesting. Thank you again for teaching me about this. I'd love to hear how your experiments turn out!
Hello man, great videos. Really helpful links. I have a question : do you pass every time series datapoint (for every single batch) through a linear layer? What is the intuition behind this "dimension augmentation" if I may call it this way ? I see a lot of Conv1D being used and am trying to understand how to perform a good embedding. I feel like most papers on TSF with transformers aren't clear on this matter.
Hi @jeanlannes4522 - thank you! You are correct: each element of each time series is embedded "individually". Conv1D may be a better embedding approach for many (possibly most/all) problems. I used the linear approach because it was easy for me to understand, as it is almost an exact analog for word embedding with PyTorch's nn.Embedding() layer. The intuition (as far I understand) is that the model learns a vector representation for each individual "datapoint". When the datapoints are words in an NLP problem these vectors are a great measure of similarity between two words. For a problem with continuous data, this doesn't make as much sense because you could just as easily measure similarity with simple distance between two points. So, when the Linear layer learns something like 0.55 and 0.56 are similar, it's not as meaningful. One could argue that Conv1D is performing a similar task, but it is considering neighboring values in the embedding process, so it could generate "smarter" embeddings like 0.55 on an "increasing trajectory/slope" is different from 0.55 on a "decreasing trajectory/slope". This is something that I may try on my own now that you mention it! Do you mind sharing any sources where this is used if you have them on hand?
@@lets_learn_transformers Thanks for your answer. There is a philosophical question that remains : if every word has a meaning, does a single datapoint of a time series have one too ? Or only a sequence of these datapoints ? Should you tokenize your time series at the datapoint scale or at a few points scale to capture a little meaning (like a pattern, increasing, flat, decreasing, volatile etc.). ? But then how do you compress your data ? The question of multivariate time series remains (what if we have p features, p > 1 ?). One could argue that some words taken alone do not have a "meaning" (it, 's, _, ', .)... It is a difficult question. To get back to what you are doing, are you training the weights of your nn.linear(1,embed size) with the big transformer backprop ? Just to make sure I understand what you are doing. I am not sure if augmenting the dimension of a single datapoint makes sense. I really think you have to work with sub-windows of the original time series. But who knows.... I believe Conv1D is interesting too. Don't know if one is allowed to leak future neighboring values. But at least the past values can add meaning to the datapoint embedding as you say "increasing trajectory" added to a given value. The first time I read it was used was in MTS-Mixers: Multivariate Time Series Forecasting via Fac- torized Temporal and Channel Mixing and Financial Time Series Forecasting using CNN and Transformer.
@@jeanlannes4522 I completely agree - thank you for a great discussion. The nn.linear weights are trained via backprop upstream from the Transformer Encoder. It is possible that this behaves ok because I'm using a very small Transformer - it is possible that the linear layer would be far too simple with a larger model. I ran some experiments on the sunspots data and found the two to be comparable - but since I'm not going in depth with hyperparameters or early stopping it's hard to tell how good the results are. Do you mind if I make a short follow-up video about this discussion? Would you like your name included / not included in the video?
nice man! it's these case studies that really generate insight. good stuff
Thank you!
Thanks for sharing
Thank you!
Great video my friend. Would love to see more stuff like this :D
Thank you!