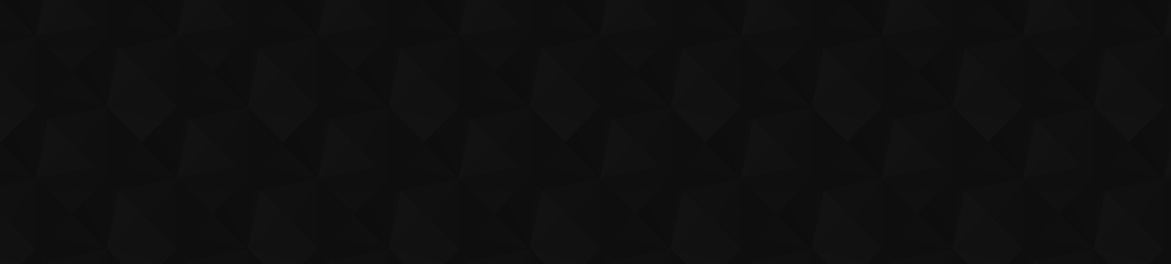
- 48
- 24 507
Lancaster CMAF
United Kingdom
Registrace 12. 03. 2020
The official channel of the Centre for Marketing Analytics and Forecasting (CMAF), Lancaster University, UK. CMAF is a pioneering centre for fundamental and applied research, leading knowledge exchange between academia and business. We have extensive experience with working in organisations, both public and private, offering research and support in sectors including telecommunications, retailing and manufacturing as well as software suppliers. Throughout the year, we host a range of events including courses and guest speakers.
CMAF FFT: Making decisions when you do not trust your forecasts
This is the seventh webinar in the Season 4 of "Friday Forecasting Talks", hosted by the Centre for Marketing Analytics and Forecasting of Lancaster University, UK.
Follow us on LinkedIn: www.linkedin.com/in/lancastercmaf/
CMAF on Twitter: LancasterCMAF
Our webpage: lancaster.ac.uk/cmaf
CMAF webinars: cmaf-fft.lp151.com/
*Content of this video*
00:00 - Introduction
02:50 - Start of the talk
05:44 - The setup (Newsvendor problem, NVP)
10:22 - Scenario 1: No forecasts
16:20 - Scenario 3: Probabilistic forecasts
20:35 - Bernoulli NVP
32:24 - Intuition for the upcoming results
35:41 - Distributionally robust solution
36:51 - Application to French electricity market
38:44 - Concluding remarks
41:00 - Q&A
The abstract: Forecasts are most often there to be used as input to decision-making. And, since forecasts are never perfect, it appears natural to ask oneself how to make decisions given their uncertainty. A large body of scientific literature has developed within probabilistic forecasting and stochastic optimisation, in response to such challenges. We will focus on the specific case of newsvendor problems, which are common within procurement, logistics and energy trading for instance. However, we will extend the discussion to the case where one does not trust the probabilistic forecasts used as input, while also being unsure about the actual loss function at hand. After introducing the Bernouilli newsvendor problem, we present more general solutions to the newsvendor problem, when one does not trust the forecasts, for both decision variable and loss function parameters. A renewable energy trading example will allow illustrating the interest of the approach.
Speaker: Pierre Pinson
Bio: Pierre Pinson is a professor at Imperial College London, Dyson School of Design Engineering. He is also a chief scientist at Halfspace, an affiliated professor at the Technical University of Denmark, and an associate research fellow at Aarhus University. He is the Editor-in-Chief of the International Journal of Forecasting. He has just received (with colleagues at Halspace and at Molslinjen in Denmark) the INFORMS Franz Edelman Award 2024 for outstanding achievements within operations research, analytics and management science.
Follow us on LinkedIn: www.linkedin.com/in/lancastercmaf/
CMAF on Twitter: LancasterCMAF
Our webpage: lancaster.ac.uk/cmaf
CMAF webinars: cmaf-fft.lp151.com/
*Content of this video*
00:00 - Introduction
02:50 - Start of the talk
05:44 - The setup (Newsvendor problem, NVP)
10:22 - Scenario 1: No forecasts
16:20 - Scenario 3: Probabilistic forecasts
20:35 - Bernoulli NVP
32:24 - Intuition for the upcoming results
35:41 - Distributionally robust solution
36:51 - Application to French electricity market
38:44 - Concluding remarks
41:00 - Q&A
The abstract: Forecasts are most often there to be used as input to decision-making. And, since forecasts are never perfect, it appears natural to ask oneself how to make decisions given their uncertainty. A large body of scientific literature has developed within probabilistic forecasting and stochastic optimisation, in response to such challenges. We will focus on the specific case of newsvendor problems, which are common within procurement, logistics and energy trading for instance. However, we will extend the discussion to the case where one does not trust the probabilistic forecasts used as input, while also being unsure about the actual loss function at hand. After introducing the Bernouilli newsvendor problem, we present more general solutions to the newsvendor problem, when one does not trust the forecasts, for both decision variable and loss function parameters. A renewable energy trading example will allow illustrating the interest of the approach.
Speaker: Pierre Pinson
Bio: Pierre Pinson is a professor at Imperial College London, Dyson School of Design Engineering. He is also a chief scientist at Halfspace, an affiliated professor at the Technical University of Denmark, and an associate research fellow at Aarhus University. He is the Editor-in-Chief of the International Journal of Forecasting. He has just received (with colleagues at Halspace and at Molslinjen in Denmark) the INFORMS Franz Edelman Award 2024 for outstanding achievements within operations research, analytics and management science.
zhlédnutí: 169
Video
CMAF FFT: Forecasting with the 2nd generation of AI
zhlédnutí 109Před měsícem
This is the sixth webinar in the Season 4 of "Friday Forecasting Talks", hosted by the Centre for Marketing Analytics and Forecasting of Lancaster University, UK. Follow us on LinkedIn: www.linkedin.com/in/lancastercmaf/ CMAF on Twitter: LancasterCMAF Our webpage: lancaster.ac.uk/cmaf CMAF webinars: cmaf-fft.lp151.com/ *Content of this video* 00:00 - Introduction 01:43 - Start of th...
Business Forecasting Principles: 04. Forecast Improvement: What, When, How?
zhlédnutí 309Před 4 měsíci
In this educational video in the series of "Business Forecasting Principles", Dr. Stephan Kolassa (our Shakespeare of forecasting) discusses how the improvements in forecasting process can be achieved, in what dimensions and how they translate to specific decisions. *Content of the video* 00:00 - Introduction 00:47 - What is a "good" forecast(ing process)? 02:30 - Accuracy & Business value 05:4...
CMAF FFT: Predicting emergency admissions to hospital specialties using real-time data
zhlédnutí 91Před 5 měsíci
This is the fifth webinar in the Season 4 of "Friday Forecasting Talks", hosted by the Centre for Marketing Analytics and Forecasting of Lancaster University, UK. Follow us on LinkedIn: www.linkedin.com/in/lancastercmaf/ CMAF on Twitter: LancasterCMAF Our webpage: lancaster.ac.uk/cmaf CMAF webinars: cmaf-fft.lp151.com/ *Content of this video* 00:00 - Introduction 01:25 - Start of th...
CMAF FFT:A new classification scheme for trend/seasonal demand forecasting and planning applications
zhlédnutí 85Před 7 měsíci
This is the fourth webinar in the Season 4 of "Friday Forecasting Talks", hosted by the Centre for Marketing Analytics and Forecasting of Lancaster University, UK. Follow us on LinkedIn: www.linkedin.com/in/lancastercmaf/ CMAF on Twitter: LancasterCMAF Our webpage: lancaster.ac.uk/cmaf CMAF webinars: cmaf-fft.lp151.com/ DISCLAIMER: In this talk, we had technical issue, due to which ...
CMAF FFT: Why you should care about exponential smoothing
zhlédnutí 410Před 8 měsíci
This is the third webinar in the Season 4 of "Friday Forecasting Talks", hosted by the Centre for Marketing Analytics and Forecasting of Lancaster University, UK. Follow us on LinkedIn: www.linkedin.com/in/lancastercmaf/ CMAF on Twitter: LancasterCMAF Our webpage: lancaster.ac.uk/cmaf CMAF webinars: cmaf-fft.lp151.com/ Link to the survey of Carlos Calderon mentioned around 3 minutes...
CMAF FFT: Hierarchical forecasting for manufacturing
zhlédnutí 185Před 9 měsíci
This is the second webinar in the Season 4 of "Friday Forecasting Talks", hosted by the Centre for Marketing Analytics and Forecasting of Lancaster University, UK. Follow us on LinkedIn: www.linkedin.com/in/lancastercmaf/ CMAF on Twitter: LancasterCMAF Our webpage: lancaster.ac.uk/cmaf CMAF webinars: cmaf-fft.lp151.com/ *Content of this video* 00:00 - Introduction 02:30 - Start of t...
CMAF FFT: Common Forecasting Methods and The Mindset Behind Forecasting
zhlédnutí 384Před 10 měsíci
This is the first webinar in the Season 4 of "Friday Forecasting Talks", hosted by the Centre for Marketing Analytics and Forecasting of Lancaster University, UK. Follow us on LinkedIn: www.linkedin.com/in/lancastercmaf/ CMAF on Twitter: LancasterCMAF Our webpage: lancaster.ac.uk/cmaf CMAF webinars: cmaf-fft.lp151.com/ *Content of this video* 00:00 - Introduction 03:20 - Start of th...
Business Forecasting Principles: 03. Judgment in Forecasting
zhlédnutí 449Před rokem
In this educational video in the series of "Business Forecasting Principles", Prof. Robert Fildes discusses the role of judgment in forecasting, touching upon the main problems with it and what can be done to make the best use of it. *Content of the video* 00:00 - Introduction 02:45 - Quantitative vs judgmental forecasting 06:40 - The process of forecasting 07:53 - Why is judgment so much used?...
Business Forecasting Principles: 02. Choice of Forecasting Approach
zhlédnutí 622Před rokem
In this educational video in the series of "Business Forecasting Principles", Prof. John E. Boylan starts a discussion of the question "How to forecast?" with a broad overview of forecasting approaches and when they can be used. *Content of the video* 00:00 - Introduction 02:44 - Types of forecasting approaches 04:45 - Situations that favour scientific methods 06:03 - Short data histories 08:19...
Lancaster CMAF introduction by Robert Fildes
zhlédnutí 113Před rokem
Robert Fildes explains why he founded the Lancaster Centre for Marketing Analytics and Forecasting, what the agenda of the centre is and what problems it is interested in.
CMAF FFT: Applying Forecasting Techniques to Healthcare Data
zhlédnutí 311Před rokem
This is the sixth webinar in the 3 Season of "Friday Forecasting Talks", hosted by Centre for Marketing Analytics and Forecasting of Lancaster University, UK. Follow us on LinkedIn: www.linkedin.com/in/lancastercmaf/ CMAF on Twitter: LancasterCMAF Our webpage: lancaster.ac.uk/cmaf CMAF webinars: cmaf-fft.lp151.com/ *Content of this video* 00:00 - Introduction 00:10 - Presentation by...
CMAF FFT: Intermittent Demand Forecasting
zhlédnutí 910Před rokem
This is the fifth webinar in the 3 Season of "Friday Forecasting Talks", hosted by Centre for Marketing Analytics and Forecasting of Lancaster University, UK. Follow us on LinkedIn: www.linkedin.com/in/lancastercmaf/CMAF on Twitter: LancasterCMAF CMAF webinars: cmaf-fft.lp151.com/ Video mentioned in the webinar: czcams.com/video/m_mI5SCjiYQ/video.html *Content of this video* 00:00 -...
Business Forecasting Principles: 01. Introduction to Forecasting
zhlédnutí 2,1KPřed rokem
In this first educational video in the series of "Business Forecasting Principles", Prof. John E. Boylan explains basics of forecasting, starting from the main misconceptions, and then moving to the discussion of main questions in forecasting: "What and Why and When and How and Where and Who..?" *Content of the video* 00:00 - Introduction 00:44 - Misconceptions in forecasting 03:30 - What's the...
CMAF FFT: Machine Learning forecasts - confirmation bias or value add?
zhlédnutí 191Před rokem
This is the fourth webinar in the 3 Season of "Friday Forecasting Talks", hosted by Centre for Marketing Analytics and Forecasting of Lancaster University, UK. Follow us on LinkedIn: www.linkedin.com/in/lancastercmaf/ CMAF on Twitter: LancasterCMAF CMAF webinars: cmaf-fft.lp151.com/ *Content of this video* 00:00 - Introduction 02:41 - Presentation by Anne-Flore Elard 08:50 - When to...
CMAF FFT: The role and challenges of forecasting for circular economic operations
zhlédnutí 132Před rokem
CMAF FFT: The role and challenges of forecasting for circular economic operations
CMAF FFT: When and what not to forecast?
zhlédnutí 296Před rokem
CMAF FFT: When and what not to forecast?
Introduction of Lancaster Centre for Marketing Analytics and Forecasting members
zhlédnutí 603Před rokem
Introduction of Lancaster Centre for Marketing Analytics and Forecasting members
CMAF FFT: Alternatives to judgmental forecast adjustments: a retail case study
zhlédnutí 187Před rokem
CMAF FFT: Alternatives to judgmental forecast adjustments: a retail case study
CMAF FFT: Making your model generalise better: Cross-validation and data augmentation
zhlédnutí 186Před 2 lety
CMAF FFT: Making your model generalise better: Cross-validation and data augmentation
CMAF FFT: On performance of temporal aggregation in time series forecasting
zhlédnutí 317Před 2 lety
CMAF FFT: On performance of temporal aggregation in time series forecasting
CMAF FFT: A new taxonomy of vector exponential smoothing and its application to seasonal time series
zhlédnutí 147Před 2 lety
CMAF FFT: A new taxonomy of vector exponential smoothing and its application to seasonal time series
CMAF FFT: Bridging the gap between forecast and business value
zhlédnutí 551Před 2 lety
CMAF FFT: Bridging the gap between forecast and business value
CMAF FFT: Using IoT Data at the Point-of-Consumption for Demand Forecasting and Inventory Management
zhlédnutí 382Před 2 lety
CMAF FFT: Using IoT Data at the Point-of-Consumption for Demand Forecasting and Inventory Management
CMAF FFT: Human vs machine: do practitioners trust forecasting algorithms?
zhlédnutí 148Před 2 lety
CMAF FFT: Human vs machine: do practitioners trust forecasting algorithms?
CMAF FFT: How traders predict prices and liquidity in the electricity market
zhlédnutí 6KPřed 2 lety
CMAF FFT: How traders predict prices and liquidity in the electricity market
CMAF FFT: Are Target Service Levels Achieved Effectively and Efficiently?
zhlédnutí 136Před 2 lety
CMAF FFT: Are Target Service Levels Achieved Effectively and Efficiently?
CMAF FFT: Best Practices for Supply Chain Demand Forecasting
zhlédnutí 621Před 2 lety
CMAF FFT: Best Practices for Supply Chain Demand Forecasting
CMAF FFT: Long-Term Forecasting for Policymaking with Structured Analogies
zhlédnutí 137Před 2 lety
CMAF FFT: Long-Term Forecasting for Policymaking with Structured Analogies
CMAF FFT: Identifying sequential changes in mean and variance within more complex model structures
zhlédnutí 220Před 2 lety
CMAF FFT: Identifying sequential changes in mean and variance within more complex model structures
What broker let’s you trade electricity?
None
Which platforms would you recommend for trading the US electricity market?
𝓟Ř𝔬𝓂𝔬𝐒ϻ 😥
Have there been any news on when the paper is going to be released?
Hi Lukas, As far as we know, the paper hasn't been even submitted to a journal yet.
𝔭𝔯𝔬𝔪𝔬𝔰𝔪 🎊
Wonderful, are there any r-code-examples for augmentation available?
Highly informative video. How do manage to incorporate covid-19 in ml model? What's the correct strategy to model covid-19 effects on revenue/sale forecast of household products?
Thanks for sharing the session.Would like to know is there any data analytics solution i.e. can be used to pull best bid/ask quotes in custom time interval of a day?, please let me know
Hi Karthikeyan, You can ask Dmirtii directly on LinkedIn: www.linkedin.com/in/dishutin - he would be glad to discuss the topic.
Thank you. Very useful and John is master in this field. Hope to see more technical videos in future :)
Thank you Team. It will be awesome if Stephen Kolassa can take a live project in Forecasting done in Retail using R. All of us who wants to learn will pay and watch it. It will be awesome if this happens
This is just brilliant. Thanks a lot to Stephan for pointing out so many important facts about point forecasting that are - for so many reason I don't fully understand - mostly completely ignored by the forecasting community despite their importance.
Thank you for this video. It will be great if Stephan can make some more videos on Time series forecasting.
Hi Vikrant, we have another video with Stephan on the topic of Accuracy measures: czcams.com/video/pD_yEmEQCeo/video.html We are thinking of making more content together with him.
@@lancastercmaf Thank you Team. These videos will be so helpful for people like us who wants to lean forecasting from the best. Again Thank you
Dear speaker your conversation is disappeared could you appear it
Is there a way to get these slides?
All the slides from CMAF FFT are available here: github.com/lancastercmaf/FFT
Hi! Thanks for sharing. Are the slides uploaded somewhere?
Hi Nikolaos, yes they are, here: github.com/lancastercmaf/FFT
P.S. Here's a good citation from (Goodwin, 2018, p. 54): "If you do want to perform FVA across products, then researchers recommend a measure called the average relative MAE (see the Davydenko and Fildes reference at the end of the chapter). This has not yet been implemented in commercial software." , see my previous comment for the references. *Source:* Goodwin, P. (2018). Profit from your forecasting software: A best practice guide for sales forecasters. Wiley and SAS Business Series. John Wiley & Sons, Inc., Hoboken, New Jersey.
Hi, guys! At 17:45 you talk about error metrics: 1) Importantly, it must be (Davydenko and Fildes, 2013), the year is 2013, not 2011 : Davydenko, A., & Fildes, R. (2013). Measuring forecasting accuracy: The case of judgmental adjustments to SKU-level demand forecasts. _International Journal of Forecasting_ , 29(3), 510-522 An adapted version of (Davydenko and Fildes, 2013) was published in this book: Davydenko, A., & Fildes, R. (2016). Forecast Error Measures: Critical Review and Practical Recommendations. In _Business Forecasting: Practical Problems and Solutions_ . John Wiley & Sons. 2) *FVA* you defined at 17:45 is exactly the *AvgRelMAE* from (Davydenko and Fildes, 2013). 3) *Bias* you defined at 17:45 is exactly the *AvgRelAME* proposed in the followng Ph.D. thesis on page 64: Davydenko, A. (2012). _Integration of judgmental and statistical approaches for demand forecasting: Models and methods_ (doctoral dissertation). Lancaster University, UK, doi.org/10.13140/RG.2.2.31788.62083 I really would appreciate it if you could cite (Davydenko, 2012, p.64) when you use the *AvgRelAME* for measuring and reporting bias. This metric was proposed in (Davydenko, 2012), I used my time, skills, and efforts to develop this metric for you. You are familiar with the source, so a proper citation is really required here. Thank you for your attention.
Additional responses from Sarah Darin to the questions asked during the webinar: Q: Would you recommend using events to help explain the big swings and combine that with domain knowledge with overrides? A: Yes, using a combination of modeling techniques with judgment is an excellent approach. Q: Do you recommend using ensemble forecasting models in dynamic regression models? A: Possibly, based on the evidence that ensemble approaches are effective. That said, I am more inclined to use something like Extreme Gradient Boosting as an ensemble approach as opposed to iterative Dynamic Regression. FYI, we are including Machine Learning methods in the next version of Forecast Pro, due out later this year. Q: How should we deal with the event factor? e.g. after the pandemic more and more people will choose online shopping, change their purchasing behaviour. A: I think this is inherent in the "new normal" that we discussed. Instead of an event, this really should be dealt with as permanent shift in the level. Extrapolative methods, such as exponential smoothing, should automatically account for a new level like this. Q: Where can I get more orientation on how to work with Forecast Pro dynamic regression modelling, when the automatic dynamics does not work well? (Ljung box parameter is not respected, for example) A: We are happy to work with you - please reach out to me at sdarin@forecastpro.com. We do provide ACF/PACF/error plots to guide this decision, making so that you can specify custom dynamics that have an appropriate Ljung Box test. A: Can you do forecasting in a "group" level e.g. a lipstick family where x% = Red, y% = brown, ... so 1 number at group level is then split per % to the lower levels. This would be done for forecasts 3+ months out. Within 3 months, we will go automatically to the sku's per color that could be maintained in detail. Do you have this functionality? A: You can forecast at a group level with a topdown modifier at any group level. We strongly encourage users to use approaches like this since very often it increases forecast accuracy. However, we do not let you specify a different model starting a given point in the forecast horizon - you need to choose sku level or group level. To achieve the forecast you describe, I recommend using the sku level forecast first, exporting those forecasts, reading those forecasts back into the project as external forecasts and then using the group level forecasts in the project. In the override grid, you can easily specify that the "external" sku forecasts be used for the first 3 months. Please take a look at www.forecastpro.com/resources/webinars/ where, if you scroll down a bit, you will find a webinar called "Effective Strategies for Forecasting a Product Hierarchy". Also feel free to reach out with questions sdarin@forecastpro.com. Q: Any insights on toll road traffic demand? Differences in short and long term? How to address possible permanent impacts? A: I certainly don't have a lot of domain knowledge, but I certainly think that some of the Google Mobility data metrics (hours spent at work, home etc.) would be helpful here. In terms of a permanent shift, a level shift is possible in dynamic regression, but I would be inclined to try and account for the drivers of the shift and then explore long run scenarios for those shift drivers (again things like google mobility, metrics that track working at home etc.) to generate a longer run forecast.
Very good overview of forecasting and demand planning. Thanks for sharing.