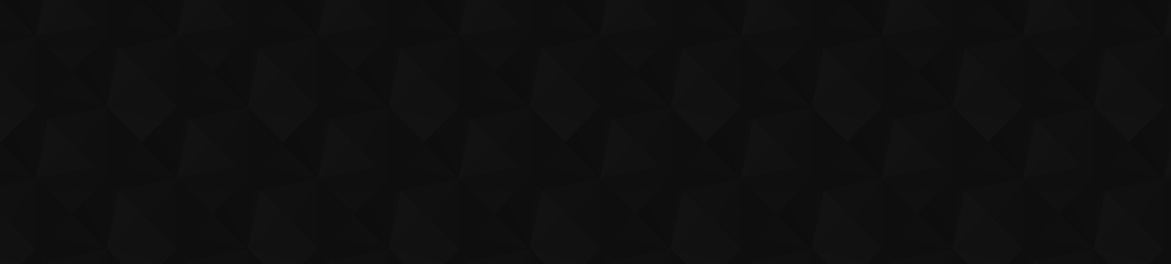
- 485
- 484 605
KDD2017 video
United States
Registrace 23. 06. 2017
KDD 2017 is the 23rd SIGKDD Conference on Knowledge Discovery and Data Mining, one of the world’s largest and best Data Science conferences. Started in 1989, KDD is the oldest & largest data mining conference worldwide. We pioneered “Big Data”, “Data Science”, and “Predictive Analytics” solutions before these names even existed - some of the first & most highly cited research papers on these topics were published in our conference. Other notable innovations that originated in our conference include crowd sourcing; large scale data mining competitions with over 10,000 participants, personalized advertising, e.g., on Google, graph mining algorithms that power Facebook & LinkedIn, and recommender systems used by Netflix, Amazon, etc. After 25 years and an explosive growth in this industry, we are still the home for the latest cutting-edge research in these topics. Even today, the technology & research discussed at our conference is often 1-3 years ahead of any other conference!
A/B Testing in Networks with Adversarial Members
Author:
Kaleigh Clary, Department of Computer Science, University of Massachusetts Amherst
Abstract:
Many researchers attempt to study the effects of interventions in network systems. To simplify experimental design and analysis in these environments, simple assumptions are made about the behavior of its members. However, nodes may not respond to treatment, or may respond maliciously. These adversarial nodes influence treatment topology by preventing or altering the expected network effect, but may not be known or detectable. We characterize the influence of adversarial nodes and the bias these nodes introduce in average treatment effect estimates. In particular, we derive expressions for the bias induced in average treatment effect using the linear estimator from Gui et al (2015). In addition to theoretical bounds, we empirically demonstrate estimation bias through experiments on synthetically generated networks. We consider both the case in which adversarial nodes are dispersed randomly through the network and the case where adversarial node placement is targeted to the highest degree nodes. Our work demonstrates that peer influence makes causal estimates on networks susceptible to the actions of adversaries, and specific network structures are particularly vulnerable to to adversarial responses.
More on www.kdd.org/kdd2017/
KDD2017 Conference is published on videolectures.net/
Kaleigh Clary, Department of Computer Science, University of Massachusetts Amherst
Abstract:
Many researchers attempt to study the effects of interventions in network systems. To simplify experimental design and analysis in these environments, simple assumptions are made about the behavior of its members. However, nodes may not respond to treatment, or may respond maliciously. These adversarial nodes influence treatment topology by preventing or altering the expected network effect, but may not be known or detectable. We characterize the influence of adversarial nodes and the bias these nodes introduce in average treatment effect estimates. In particular, we derive expressions for the bias induced in average treatment effect using the linear estimator from Gui et al (2015). In addition to theoretical bounds, we empirically demonstrate estimation bias through experiments on synthetically generated networks. We consider both the case in which adversarial nodes are dispersed randomly through the network and the case where adversarial node placement is targeted to the highest degree nodes. Our work demonstrates that peer influence makes causal estimates on networks susceptible to the actions of adversaries, and specific network structures are particularly vulnerable to to adversarial responses.
More on www.kdd.org/kdd2017/
KDD2017 Conference is published on videolectures.net/
zhlédnutí: 459
Video
Learning to Make Stuff
zhlédnutí 127Před 6 lety
Author: Mark Riedl, Georgia Institute of Technology More on www.kdd.org/kdd2017/ KDD2017 Conference is published on videolectures.net/
Cultural Creativity
zhlédnutí 169Před 6 lety
Author: Nick Montfort, Writing and Humanistic Studies, Center for Future Civic Media, Massachusetts Institute of Technology, MIT More on www.kdd.org/kdd2017/ KDD2017 Conference is published on videolectures.net/
Stacked Ensemble Models and Data Science Competitions
zhlédnutí 1,2KPřed 6 lety
Author: Funda Günes, SAS Institute Inc. More on www.kdd.org/kdd2017/ KDD2017 Conference is published on videolectures.net/
Managing Research Team Panel
zhlédnutí 109Před 6 lety
Panelists: Ivan Brugere, University of Illinois at Chicago Tim Weninger, Department of Computer Science and Engineering, University of Notre Dame Monica Anderson, College of Engineering, University of Alabama Moderator: Christan Grant, School of Computer Science, University of Oklahoma More on www.kdd.org/kdd2017/ KDD2017 Conference is published on videolectures.net/
Mentoring Session Panel
zhlédnutí 190Před 6 lety
Panelists: Mario Guajardo-Cespedes, Research at Google, Google, Inc. Jennifer Wortman Vaughan, Microsoft Research Funda Günes, SAS Institute Inc. Isabelle Moulinier, Capital One Anthony D. Joseph, Department of Electrical Engineering and Computer Sciences, UC Berkeley Moderator: Annie En-Shiun Lee, Centre for Pattern Analysis & Machine Intelligence (CPAMI), University of Waterloo More on www.kd...
Profit Maximization for Online Advertising Demand-Side Platform
zhlédnutí 571Před 6 lety
Author: Paul Grigas, Industrial Engineering and Operations Research Department, UC Berkeley Abstract: We develop an optimization model and corresponding algorithm for the management of a demand-side platform (DSP), whereby the DSP aims to maximize its own profit while acquiring valuable impressions for its advertiser clients. We formulate the problem of profit maximization for a DSP interacting...
MM2RTB: Bring Multimedia Metrics to Real-Time Bidding
zhlédnutí 96Před 6 lety
Author: Xiang Chen, Department of Computer Science, National University of Singapore Abstract: In display advertising, users’ online ad experiences are important for the advertising effectiveness. However, users have not been well accommodated in real-time bidding (RTB). is further influences their site visits and perception of the displayed banner ads. In this paper, we propose a novel computa...
Ranking and Calibrating Click-Attributed Purchases in Performance Display Advertising
zhlédnutí 838Před 6 lety
Author: Abraham Bagherjeiran, A9.com Inc. Abstract: In performance display advertising, bidders compete on behalf of advertisers for ad impressions, that is, the opportunity to display relevant ads on a publisher website. We consider bidding on behalf of online retailers who buy ad impressions hoping to realize value only from purchases attributed from clicks. The bidder has a two stage problem...
Machine Learning and Causal Inference for Advertising Effectiveness
zhlédnutí 4,2KPřed 6 lety
Author: Susan Athey, Stanford Graduate School of Business, Stanford University Abstract: This talk will review several recent methodological approaches to measuring the effectiveness of treatments, such as advertising campaigns. We will discuss methods for dealing with non-random assignment of advertisements to users, as well as methods for estimating optimal policies for assigning advertising ...
Deep & Cross Network for Ad Click Predictions
zhlédnutí 3,2KPřed 6 lety
Author: Ruoxi Wang, Institute for Computational and Mathematical Engineering, Stanford University Abstract: Feature engineering has been the key to the success of many prediction models. However, the process is nontrivial and often requires manual feature engineering or exhaustive searching. DNNs are able to automatically learn feature interactions; however, they generate all the interactions i...
Blacklisting the Blacklist in Online Advertising
zhlédnutí 162Před 6 lety
Author: Yeming Shi, Dstillery Abstract: Every day, billions of online advertising slots are bought and sold through real time bidding (RTB). In RTB, publishers sometimes reject bids to deliver ads (impressions) for some brands, due to, for example, direct deals with other brands. Publishers rarely disclose which brands they blacklist to ad buyers. Buyers bidding for a blacklisted brand waste co...
Attribution Modeling Increases Efficiency of Bidding in Display Advertising
zhlédnutí 273Před 6 lety
Author: Julien Meynet, Criteo Abstract: Predicting click and conversion probabilities when bidding on ad exchanges is at the core of the programmatic advertising industry. Two separated lines of previous works respectively address i) the prediction of user conversion probability and ii) the attribution of these conversions to advertising events (such as clicks) after the fact. We argue that att...
Data-Driven Reserve Prices for Social Advertising Auctions at LinkedIn
zhlédnutí 347Před 6 lety
Author: Kun Liu, LinkedIn Corporation Abstract: Online advertising auctions constitute an important source of revenue for search engines such as Google and Bing, as well as social networks such as Facebook, LinkedIn and Twitter. We study the problem of setting the optimal reserve price in a Generalized Second Price auction, guided by auction theory with suitable adaptations to social advertisin...
Cost-sensitive Learning for Utility Optimization in Online Advertising Auctions
zhlédnutí 429Před 6 lety
Cost-sensitive Learning for Utility Optimization in Online Advertising Auctions
Optimal Reserve Price for Online Ads Trading Based on Inventory Identification
zhlédnutí 250Před 6 lety
Optimal Reserve Price for Online Ads Trading Based on Inventory Identification
An Ensemble-based Approach to Click-Through Rate Prediction for Promoted Listings at Etsy
zhlédnutí 910Před 6 lety
An Ensemble-based Approach to Click-Through Rate Prediction for Promoted Listings at Etsy
A Hybrid Approach for Sentiment Analysis Applied to Paper
zhlédnutí 827Před 6 lety
A Hybrid Approach for Sentiment Analysis Applied to Paper
Deep Learning for Contrasting Meaning Representation and Composition
zhlédnutí 94Před 6 lety
Deep Learning for Contrasting Meaning Representation and Composition
Viscovery: A Platform for Trend Tracking in Opinion Forums
zhlédnutí 38Před 6 lety
Viscovery: A Platform for Trend Tracking in Opinion Forums
Creating Domain-Specific Sentiment Lexicons via Text Mining
zhlédnutí 902Před 6 lety
Creating Domain-Specific Sentiment Lexicons via Text Mining
Barycentric coordinates for ordinal sentiment classification
zhlédnutí 79Před 6 lety
Barycentric coordinates for ordinal sentiment classification
From Bioinformatics to Precision Medicine
zhlédnutí 218Před 6 lety
From Bioinformatics to Precision Medicine
Cost-sensitive Deep Learning for Early Readmission Prediction at A Major Hospital
zhlédnutí 158Před 6 lety
Cost-sensitive Deep Learning for Early Readmission Prediction at A Major Hospital
Improving the Prediction of Functional Outcome in Ischemic Stroke Patients
zhlédnutí 273Před 6 lety
Improving the Prediction of Functional Outcome in Ischemic Stroke Patients
Ontology-based workflow extraction from texts using word sense disambiguation
zhlédnutí 466Před 6 lety
Ontology-based workflow extraction from texts using word sense disambiguation
Coordination Event Detection and Initiator Identification in Time Series Data
zhlédnutí 108Před 6 lety
Coordination Event Detection and Initiator Identification in Time Series Data
Robust Parameter-Free Season Length Detection in Time Series
zhlédnutí 62Před 6 lety
Robust Parameter-Free Season Length Detection in Time Series
Sir, there is no youtube video on cubist model. Could you kindly make a video on cubist regression.
WHere is the source code?
Gemini 1.5 Pro: This video is about Google Vizier, a service for blackbox optimization developed by Google. Blackbox optimization is a type of optimization where the system you are trying to optimize is opaque, meaning you can only evaluate different configurations by running experiments. Vizier makes it easy to define and run these experiments, and it also provides a number of features to help you improve the efficiency of your search. Here are the key design goals of Vizier: * Ease of use: Vizier is designed to be easy to use, even for people who are not familiar with blackbox optimization. * Availability: Vizier is highly available, so you can be confident that it will be up and running when you need it. * Scalability: Vizier can scale to handle large workloads, so you can use it to optimize even the most complex systems. * Performance: Vizier uses state-of-the-art algorithms to find the best possible configuration for your system. * Flexibility: Vizier is flexible and can be used to optimize a wide variety of systems. Vizier is a powerful tool that can be used to optimize a wide variety of systems. It is easy to use, available, scalable, performs well, and flexible. If you are looking for a way to improve the performance of your system, Vizier is a great option to consider. Some of the applications of Vizier include: * Automated optimization for user interfaces or user experience * Optimizing machine learning models * Physical design problems * Robotics One interesting example of how Vizier has been used is to design chocolate chip cookies. By parameterizing the space of possible cookie recipes, Vizier was able to generate a series of cookies that were then taste-tested by humans. The results of this experiment were used to improve the Vizier algorithm.
Hello dear Sir, I have question what is the difference between vanilla-based causality and optimal Markov blanket?
Can u send me your pdf too
Such an amazing session!!!!
I think an approach like this need to be explained step-by-step and without music in the background.
How do I get the code for this method?
A really good explanation on math behind Stumpy package. I also downloaded the presentation from the UCR website. A minor thing. On slide 23, is it should be n/2 > m instead of n > m/2?
This video was perfect for where I'm at educationally, thank you. Very informative.
Really well explained ! Thank you for the lecture. Is the material presented available ?
I would like to thank you for share the presentation, but 90% of screen is the audience and not the presentation (or the presenter). It's a waste of space, information and resources.
how to compute the visual graph at 26:52 ? i don't really understand the meaning of the x and y axis
I can't believe it can be this good
I appreciate the upload. Thanks for the video!
Such neatly explained. Shows the intuition well. loved it!
This research is great. I'd be very grateful if you can share the related implementation and database.
Where is the tutorial website
Hello sir, this video is inspiring thank you. please 🙏 how can I predict unknown geographical companies locations from known geographical companies locations and map representation, have some relevant data such as population density, economic infrastructures, main roads, electrical network lines..
😴😴😴
If you take a simple time series and compute her matrice profile it will be more useful than one speech of 1 hour
Brian Christian brought me here.
Hello sir can you please tell me how to use ego algorithm if our function is not known at all
great talk ever
Crypt-O is a tool for evil. Illuminati & evil elites use it for child sex trafficking and buying/selling adrenochrome. Watch what's being revealed since the Ghislaine Maxwell indictment. Evenually, it can and will be brought down.
the sound is not good I am quite disappointed
The video sound is pretty good, beyond my imagination
audience has no sense of humor
1.5xx
Great job!
any idea where I can get the slides?
Isn't time complexity of STMP O((n-m+1)*n*logn) as you run the MASS algorithm n-m+1 times? Or is it because you can assume that n >> m therefore, n-m+1=n ?
Thanks for the detailed explanation
Interesting talk!
Just call it a voronie diagram
your only as strong as your weakest link!
can u plsssssssss send me the pdf
the previous time stamp is t-dt1 and the next time stamp is t+dt2 because the gap is irregular. it will not be correct to say t-1,t,t+1 in irregular scenario. this is what i think.
Is there a link to his other talk he mentions at the end?
wow, that's smart :O
Thank you for offering so great video :) Is the number ego-net cluster is a hyper-parameter? or is it being naturally defined according to the network structure?
Very cool video
...
Hi, what is Time complexity of co-training?
Great lecture. I loved the level of detail and examples. How can reach you for questions?
I am trying so hard to understand what he is saying.. :-( :-(.. He accent and pronunciation is very difficult to understand.
Very interesting work! Opens a whole new perspective on time series analysis!
are the slides available?
bookerystore.com/downloads/bandit-algorithms/
Which method is currently using for Android malware detection? Please reply soon