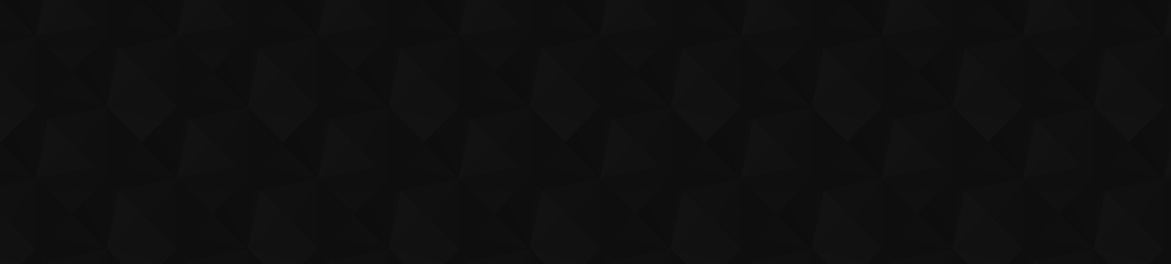
- 96
- 70 245
Feature Store Org
Registrace 16. 12. 2020
The featurestore.org community provides a forum for the international community of users and developers of Feature Store platforms and tools to share ideas and learn from each other.
The Feature Store Global Meetup is a vendor-free and safe space to discuss everything in between feature engineering, feature management, and feature usage for model training and model serving. Featurestore.org promotes data engineering and data science using many languages, including (but not limited to) Python, Scala, and Java.
Join us every month for a live virtual meetup where top experts share their best practices, new approaches, and emerging technologies to improve your ML pipeline. If you're interested to present, you can also submit a talk: featurestore.org/submit-a-talk/
The Feature Store Global Meetup is a vendor-free and safe space to discuss everything in between feature engineering, feature management, and feature usage for model training and model serving. Featurestore.org promotes data engineering and data science using many languages, including (but not limited to) Python, Scala, and Java.
Join us every month for a live virtual meetup where top experts share their best practices, new approaches, and emerging technologies to improve your ML pipeline. If you're interested to present, you can also submit a talk: featurestore.org/submit-a-talk/
WeChat - WeChat's Feature Platform For Real-Time Recommender Systems - FS Summit 23
Jin is a software engineer on WeChat's Machine Learning Platform team, where he maintains the feature compute engine for their in-house feature platform.
In his talk, he introduces WeChat’s feature platform, emphasizing their Apache Arrow based vectorized feature compute engine for low-latency online serving, and approaches for ensuring online-offline consistency and data integrity.
#featurestoresummit
In his talk, he introduces WeChat’s feature platform, emphasizing their Apache Arrow based vectorized feature compute engine for low-latency online serving, and approaches for ensuring online-offline consistency and data integrity.
#featurestoresummit
zhlédnutí: 274
Video
DAGWorks - Feature Engineering with Hamilton: Write Once Run Everywhere- FS Summit 23
zhlédnutí 244Před 10 měsíci
Elijah built large components of the simulation/trading infrastructure at Two Sigma, where he led a team to test/ensure the reliability of those simulations. Most recently he co-authored the open source library Hamilton, a general-purpose lightweight framework for building dataflows in Python. In his talk, he discusses Hamilton, a lightweight open-source framework in python that enables data pr...
Uber - Palette Metadata Journey - FS Summit 2023
zhlédnutí 195Před 10 měsíci
Paarth is a Staff Software Engineer on Uber AI’s Feature Store team and specializes in large scale distributed systems for ML Infrastructure. Nicholas is a Staff Software Engineer, TLM on Uber AI’s Feature Store team and specializes in serving data for ML models at high scale. The Uber team presents their Palette Metadata Journey and how they have evolved the Metadata systems to meet Uber’s bus...
Arize - Stop Blaming the Algorithm: It’s Your Feature Store! - FS Summit 23
zhlédnutí 128Před 10 měsíci
Claire is currently Head of ML Solutions Engineering @Arize, leading post-sales machine learning solutions engineering efforts and assisting Arize customers in integrating ML Observability into their MLOps infrastructure and workflows. In her talk, she explores strategies for preventing feature store failures that often go unnoticed, emphasizing methods to protect your models. She also demonstr...
Featureform - Feature Stores aren't about storage - FS Summit 23
zhlédnutí 114Před 10 měsíci
Simba is the founder & CEO of Featureform. He started his ML career in recommender systems where he architected a multi-modal personalization engine that powered 100s of millions of user's experiences. In his talk, he breaks down the different types of feature store architectures, with a specific focus on the virtual architecture and how it fits into a wider MLOps stack. #featurestoresummit
Fennel - Architecture of Fennel’s realtime feature platform - FS Summit 23
zhlédnutí 137Před 10 měsíci
Nikhil is the CEO - Co-Founder of @Fennel, a realtime feature store built by an ex-Facebook team. In his talk, he describes the architecture powering Fennel and the various design decisions & tradeoffs that led to this architecture. He also describes the powerful capabilities unlocked by some of Fennel’s design decisions. #featurestoresummit
H2O.ai / AT&T - Operationalizing AI at Scale with H2O Feature Store - FS Summit 23
zhlédnutí 87Před 10 měsíci
Chris is Director of Customer Solutions for FSI and Strategic Products at H2O.ai, serves as product manager for H2O Feature Store and is responsible for key client relationships with global systemically important banks and other financial institutions. Prince is an accomplished leader in the field of Data and Artificial Intelligence (AI), having made significant contributions to AT&T through hi...
Databricks - Delivering Personalized & Realtime Context for LLM - FS Summit 23
zhlédnutí 156Před 10 měsíci
Aakrati is an ML/AI Engineer working on building the Feature Store for the ML Platform at Databricks. Prior to Databricks, she has worked on distributed systems and ML Infrastructure at Google. Mingyang Ge is an ML/AI Engineer working on building the Feature Store for the ML Platform at Databricks. Before Databricks, he worked Conversational Platform at Google Assistant. In their talk, they sho...
Hopsworks - From building to using feature stores for ML systems - FS Summit 2023
zhlédnutí 529Před 10 měsíci
Fabio is VP of Engineering at Hopsworks, leading the Feature Store development team. In his talk, he shows how to apply solid software engineering and MLOps principles for change control, staged feature rollout and availability to deliver high quality features in real time for model predictions. #featurestoresummit
Uber - Uber's Risk Knowledge Platform - FS Summit 23
zhlédnutí 175Před 10 měsíci
Christopher is a ML Engineer on the Risk Applied Machine Learning team at Uber. He builds ML Infrastructure tools to assist machine learning engineers across the Risk Platform in building effective model training data for fraud and abuse problems. Jean is a senior Software Engineer in Uber's Risk Knowledge Platform team. She is a key member working on the large scale distributed self-served fea...
Feature Byte - Accelerating Data Science through Feature Platforms and Generative AI - FS Summit 23
zhlédnutí 70Před 10 měsíci
Xavier is a visionary Data Scientist and co-founder of FeatureByte, a company committed to empowering data enthusiasts and streamlining feature pipelines. Before co-founding FeatureByte, Xavier ranked as the #1 Kaggle competitor in 2012-2013 and pioneered Auto-ML at DataRobot. In his talk he explores how bringing Feature Platforms and Generative AI together can make the entire feature engineeri...
Wayfair - Wayfair’s Mercury Feature Platform - FS Summit 23
zhlédnutí 259Před 10 měsíci
Alexander is a Staff ML Scientist on Wayfair’s Marketing Science team. Previously, he led development of the Mercury feature platform, as well as several initiatives improving marketing personalization. In his talk, he describes Mercury, discusses how this has resulted in faster, cheaper, and easier to maintain ML at Wayfair, talk through the strategic trade offs of being highly-opinionated and...
Delivery Hero - Driving Efficiency & Quality through a Feature Store at Delivery Hero - FS Summit 23
zhlédnutí 168Před 10 měsíci
Breno is a seasoned ML Engineer with many years of experience in ML-driven software development. He developed and delivered two Feature Stores running in production, as well as other platform components to streamline different steps of ML lifecycle, such as model development, deployment and monitoring processes. In his talk he will discuss how the ML platform team at Delivery Hero, has develope...
Kick-off - FS Summit 2023
zhlédnutí 227Před 10 měsíci
Jim Dowling kicks off the event with an introduction on a retrospective analysis of the feature store landscape in 2023. #featurestoresummit
Bytewax - Bringing ML Online: Defining ML Features in Real-Time - FS Summit 23
zhlédnutí 247Před 10 měsíci
Zander is the CEO and founder of Bytewax. Before starting Bytewax he worked across machine learning infrastructure and data science teams at Heroku and GitHub. In his talk, he showcases how Bytewax makes it easy to add real-time capabilities to products and specifically and how to build real-time feature pipelines with streaming data in Python. #featurestoresummit
Serverless ML - LAB 06 - Real-Time Machine Learning
zhlédnutí 276Před rokem
Serverless ML - LAB 06 - Real-Time Machine Learning
Lecture 6 - Operational Machine Learning Systems; Realt-time ML
zhlédnutí 803Před rokem
Lecture 6 - Operational Machine Learning Systems; Realt-time ML
Serverless ML - Lab 05 - MLOps Versioning, Expectations and Testing,
zhlédnutí 184Před rokem
Serverless ML - Lab 05 - MLOps Versioning, Expectations and Testing,
Lecture 5 - Part 02 - Principles and Practices of MLOps
zhlédnutí 452Před rokem
Lecture 5 - Part 02 - Principles and Practices of MLOps
Lecture 5 - Part 01 - Principles and Practices of MLOps
zhlédnutí 621Před rokem
Lecture 5 - Part 01 - Principles and Practices of MLOps
Serverless ML - LAB 04 - Serverless UIs with Gradio and Streamlit& Credit Card Fraud Batch UI.
zhlédnutí 403Před rokem
Serverless ML - LAB 04 - Serverless UIs with Gradio and Streamlit& Credit Card Fraud Batch UI.
Lecture 04 - Serverless User Interfaces forMachine Learning Systems
zhlédnutí 450Před rokem
Lecture 04 - Serverless User Interfaces forMachine Learning Systems
Serverless ML - LAB 03 - Training Pipelines & Batch Inference Pipelines for Credit Card Fraud
zhlédnutí 431Před rokem
Serverless ML - LAB 03 - Training Pipelines & Batch Inference Pipelines for Credit Card Fraud
Panel 02 - Data Quality & Feature Stores - FS Summit 2022
zhlédnutí 188Před rokem
Panel 02 - Data Quality & Feature Stores - FS Summit 2022
Arize - Feature Store Observability: What it is, and why it matters - FS Summit 2022
zhlédnutí 171Před rokem
Arize - Feature Store Observability: What it is, and why it matters - FS Summit 2022
Uber - Accelerating ML at Uber with the Palette Feature Store - FS Summit 2022
zhlédnutí 1,6KPřed rokem
Uber - Accelerating ML at Uber with the Palette Feature Store - FS Summit 2022
Airbnb - Introducing Zipline: An Open Source Feature Engineering Platform - FS Summit 2022
zhlédnutí 1,2KPřed rokem
Airbnb - Introducing Zipline: An Open Source Feature Engineering Platform - FS Summit 2022
Panel 01 - APIs for Feature Stores - FS Summit 2022
zhlédnutí 207Před rokem
Panel 01 - APIs for Feature Stores - FS Summit 2022
Linkedin - Feathr: An Enterprise-Grade, High Performance Feature Store - FS Summit 2022
zhlédnutí 1,1KPřed rokem
Linkedin - Feathr: An Enterprise-Grade, High Performance Feature Store - FS Summit 2022
Hopsworks - Hopsworks Feature Store after 4 years: Lessons learned and what's next - FS Summit 2022
zhlédnutí 1,2KPřed rokem
Hopsworks - Hopsworks Feature Store after 4 years: Lessons learned and what's next - FS Summit 2022
great video, thanks for sharing
Thank you very much <3
Does getML expose a C/C++ API directly? Tried to download it yesterday but the install broke with a 'wheels' error. Probably due to my having Python version greater than 3.11. Will wait until a later update.
Hi sir, hope you are doing well! I got a problem with the Model Registry. This piece of code: iris_model.save(model_dir), give me a HTTP 500 error, i am unable to save the model in the model registry
Also is there any other upcoming events for feature store? Any newsletter or forum would be helpful as well. Thanks!
Hi! For news on upcoming events and the next Feature Store Summit you can join the slack channel and newsletter on our page here: www.featurestore.org/
hello, great work. do we have any topic for KPIs for Feature store?
how can it use the write api when you ingest data with kafka or spark?
If you have a Spark application (that reads from Kafka, for example), it can write the data as a Spark DataFrame to Hopsworks. See more details in the docs: docs.hopsworks.ai/3.3/user_guides/fs/feature_group/create/
Hi Jim, does deep learning need a feature store. It might be a premature question. Just wanted to clarify with you. I read that deep learning doesn't require feature engineering.
Deep learning does 'feature engineering' for you, in some sense. The inputs to a deep learning model are numerical feature values - so you have to encode categorical features. You may need to 'normalize/scale' your numerical input features to improve convergence for your DNN. You should also batch your input features to improve GPU utilization (assuming you are doing training/inference on a GPU). Then, you will need enough input samples for a DNN to outperform a tree-based model.
@@jimdowling1275 .so still we need a feature for DL to store the numerical values?
Good start! 🔥 I hope to finish this fantastic course! 💜
Great content and great presentation and visual in this video and you covered a LOT. Thanks for putting this together.
Hi Jim, a few questions. 1. is the backfill of historical data an one time activity? 2. is feature store required for all models. for example if a client provides all the details (features) required for a prediction , is a feature store required still?. i agree it will be a simple model may be used in research scenarios.
1. typically yes, you only backfill a feature group with historical data once. If you create a new version of a feature group, you may need to backfill that one using the same historical data, though. 2. The feature store is required for online models that need historical or contextual features that are not available in the online application. Otherwise (if you only have batch), it's optional - although I think it makes pipelines easier and helps you break down the monolithic end-to-end ML pipeline into easier to manage, decoupled feature/training/inference pipelines.
Thanks @@jimdowling1275 for clarifying.
Great lectures and content, team! Heads up, you may want to reorder the playlist. Right now, the last three videos are at the top in reverse chronological order and the rest of the videos follow in chronological order. That makes it hard to watch the videos in order using the playlist. For YT playlists, it's generally best to have them in the intended view order -- here, chronological.
Hey Charles, thanks for the heads-up! The playlist order should be fixed now! 😊
@@featurestoreorg awesome, thanks!
Which python version should we use in our environment?
Python 3.7 to 3.9 are supported by hopsworks.
@@jimdowling1275 Thank you. 😁
Great lectures and labs. Question on `cc_features.py` from 17:00. Should it not be ` df_std = pd.DataFrame(cc_group.std())` and `df_count = pd.DataFrame(cc_group.count())`?
What are the differences between transformed and untransformed features?
They are covered in both slides 22-24 of this lecture and also in lecture 3 (drive.google.com/file/d/1XhfnH7DzwDqQKS6WxDVqWFFas0fi_jnJ/view). Model-specific transformations of features are when you change feature values to either (1) make the data compatible for your model - e.g., transform a stringified categorical variable into a numerical representation (e.g., one-hot encoded) and (2) to improve model performance (e.g., normal a numerical feature for a deep learning model).
@@jimdowling1275 Thank you, I understand now.
@@jimdowling1275 Ah I see. Thank you. I am currently in Lecture 02.
@@jimdowling1275 Anyway to reshare the slides?
great course
Hi , im having issues installing housework on my Mac error: legacy-install-failure × Encountered error while trying to install package. ╰─> confluent-kafka
@Moritz Meister thank you
This is very helpful! Going to start following along with the course
We now added timestamps for all the sessions. Enjoy! 🙂 26:38 - Kick-off 48:47 - Uber 01:14:33 - Hopsworks 01:38:42 - Doordash 02:04:25 - Linkedin/Microsoft 02:28:50 - Panel 01: APIs for Feature Stores 03:02:38 - Disney Streaming 03:28:19 - Airbnb 03:55:31 - Shiba 04:19:18 - Arize 04:43:21 - Panel 02: Data Quality & Feature Stores 05:17:43 - Stitch Fix 05:39:23 - AFCU 05:58:39 - Scribble Data 06:09:39 - Featureform 06:18:42 - Panel 02: How to make the feature store part of the productionalization of ML models 06:54:53 - AtScale 07:17:13 - dotData 07:27:37 - Open DataOps Foundation 07:45:55 - Sumatra 07:59:37 - UC Berkeley 08:09:10 - 4Paradigm
Great lab! One question: How to create a feature view with only a part of the data (say 100 rows) from the feature store? What should be the query for that?
The feature view doesn't contain any rows. It's just a grouping of features. When you create training data with a feature_view, then it creates rows of data. You can add filters when creating either the feature_view or the training_data to only return a subset of the data. Read more here: docs.hopsworks.ai/3.0/user_guides/fs/feature_view/query/#filter
@@jimdowling1275 Thank you
22:30 agenda
Microsoft: 2:04:00
Am just Happy to be here!! Exactly what I need :)
Great course. This is exactly what I was looking for after doing several ML/DL courses. Thank you !!
I am not able to see the option to create hopworks API when I login. Anyone facing the same issue?
Try logging out and in again. There was an issue today for people who registered with github.
@@jimdowling1275 that worked! thanks for the timely update.
can't wait for tomorrow!
Can we have the recommended data used in the databricks tutorial? NONE of the code examples in your tutorials runs due to lack of data, it’s a HORRIBLE experience for people trying to learn the technology.
How to have a live feature table
how to do backfill?
Are the slides available anywhere?
thanks for the vid wow, I found it's rather impossible to use python in snowflake, seems it's just a name there to satisfy customers rather than being functional quite disappointed
where to get notebook this guy demo? It's great sharing. Look forward to use AWS FS to speed up model developing. Thanks
How do you handle artifact references from local, transitioning to cloud? Is there an assumption from the DS to make the artifact accessible from both environments? Also does SAME handle steps across multiple notebooks?
super cool